Responsible AI – Reliability & Safety
As discussed in our first blog post on Fairness in this series on Responsible AI principles, we’ve spoken with many prospects and customers about Responsible AI over the years and always welcome (and encourage) debate in these conversations.
Previously we had explained how there are high-level “lenses” through which to develop, assess, and deploy products and services using AI, and these principles are components of a Responsible AI framework.
Fairness, Reliability & Safety, Privacy & Security, Inclusiveness, Transparency, and Accountability are the core principles we’ll be discussing in this series of blogs. Our first blog explored Fairness in more depth. This blog post will be about Reliability and Safety.
What is Reliability & Safety in a Responsible AI framework?
Reliability and safety in a Responsible AI framework refer to the assurance that AI systems will perform consistently, accurately, and safely across a variety of conditions and in the face of unexpected situations. These characteristics are crucial to building trust with users and ensuring that the technology does not cause harm. Here’s a deeper look at each aspect:
- Reliability: This involves the consistent performance of the AI system across time and various operational contexts. It means that the system should function as intended and deliver predictable, accurate results. Reliability also covers the system's ability to handle edge cases or rare situations that may not have been frequently observed in the training data.
- Safety: This refers to the system's capability to operate without causing any harm to users or the environment. Safety in AI systems includes preventing physical harm, protecting data privacy, and ensuring security against attacks or manipulations. It also involves the system’s ability to handle errors or failures gracefully without catastrophic outcomes.
When we work with customers on AI projects, we often use the Responsible AI dashboard in Azure Machine Learning, to assess reliability and safety, including through comprehensive error analysis. The tools we deploy allow developers and data scientists to analyze and understand model failures as well as identify high-risk cohorts. By understanding where and how the AI model fails, developers can work to improve the model’s reliability. This analysis helps in identifying patterns or conditions under which the model underperforms. Spotting subsets of data (cohorts) where the error rate is unusually high compared to the overall performance is particularly important for ensuring that the model does not unfairly underperform for specific demographic groups or under specific conditions, which ties directly into the safety and fairness of the AI system.
Incorporating reliability and safety within the Responsible AI framework ensures that AI systems are trustworthy and can be confidently deployed in real-world applications, knowing they will perform as expected and mitigate risks of harm.
Contextual Examples of Reliability & Safety
The application of Reliability and Safety principles can vary depending on how the data is being used and the specific products being designed. The context in which AI systems are deployed heavily influences the specific reliability and safety requirements.
Consumer Applications:
- Reliability: Consumer apps like recommendation systems (e.g., for shopping or media streaming) must reliably handle diverse user inputs and preferences without failure.
- Safety: Safety concerns might include safeguarding user data privacy and ensuring the system does not generate harmful or inappropriate content.
Healthcare:
- Reliability: In healthcare, AI tools used for diagnosis or treatment recommendations must provide highly reliable outputs, given the direct impact on patient health.
- Safety: Safety is critical to avoid misdiagnoses or incorrect treatment plans. This includes ensuring the AI system can handle anomalous medical data and integrate smoothly with other clinical systems.
Automotive / Autonomous Vehicles:
- Reliability: For self-driving cars, reliability means consistent performance under various driving conditions and environments.
- Safety: Safety is paramount to prevent accidents. This includes the ability of the system to make safe decisions in unexpected road conditions or system failures.
Financial Services:
- Reliability: Financial models (e.g., for credit scoring or fraud detection) need to reliably process transactions and accurately detect anomalies without false positives that could block legitimate transactions.
- Safety: Ensuring the security of financial data and preventing manipulative practices that could lead to financial loss for users or institutions.
Industrial Automation:
- Reliability: In industrial settings, AI systems must reliably control or predict machinery behavior over long periods.
- Safety: Safety here includes preventing accidents and ensuring that AI-driven machinery can identify and react to unsafe operational conditions.
AI in Governance and Public Sector:
- Reliability: AI used in public services (e.g., allocation of resources, predictive policing) must reliably handle data and deliver consistent outcomes across diverse populations.
- Safety: It is crucial to ensure that such systems do not reinforce biases or lead to unfair treatment of certain groups.
Each application area may require different techniques and considerations for testing, monitoring, and improving the reliability and safety of AI systems. For example, robustness to input variability, resilience to adversarial attacks, and transparency in decision-making processes might be emphasized differently depending on the application. Furthermore, regulatory requirements can also influence the specific safety and reliability measures that need to be implemented.
Summary
The challenge in Responsible AI is to navigate the application for principles to develop AI systems that are safe and reliable in a comprehensive sense. This often requires a multi-disciplinary approach that incorporates ethical, legal, technical, and social perspectives. Transparency, accountability, and ongoing engagement with stakeholders are also crucial to address these complexities effectively.
This is why having a framework, a well-rounded cross-disciplinary team involved, and a drive for an internal Center of Excellence is so important. It’s why we advise, support, engage, and champion the use of Responsible AI frameworks in organizations. If your organization would like to discuss this further, please contact us!
To read our Responsible AI White paper view here: https://infusedinnovations.com/responsible-ai
Stay connected. Join the Infused Innovations email list!
Share this
You May Also Like
These Related Posts
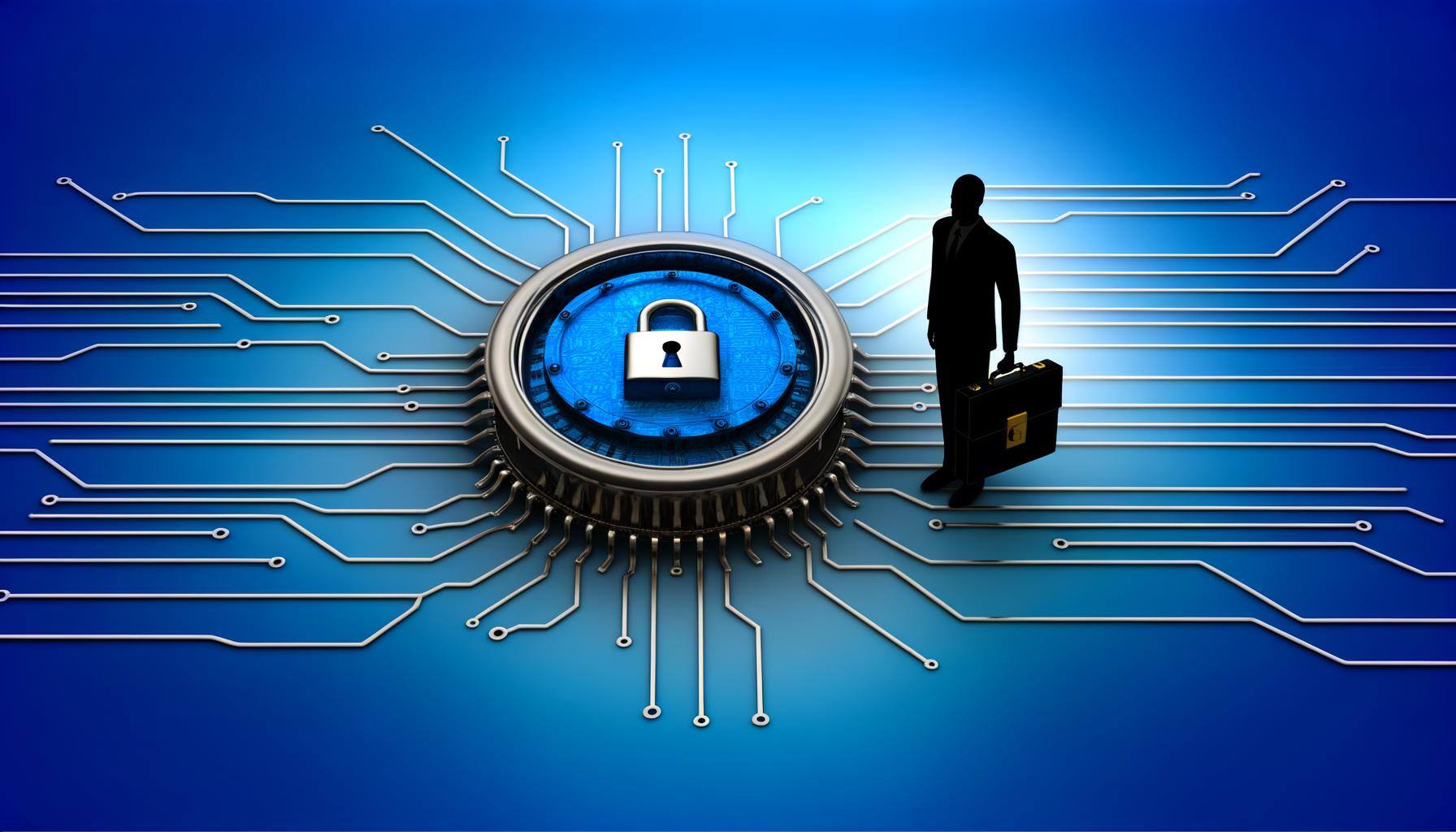
Responsible AI – Privacy & Security
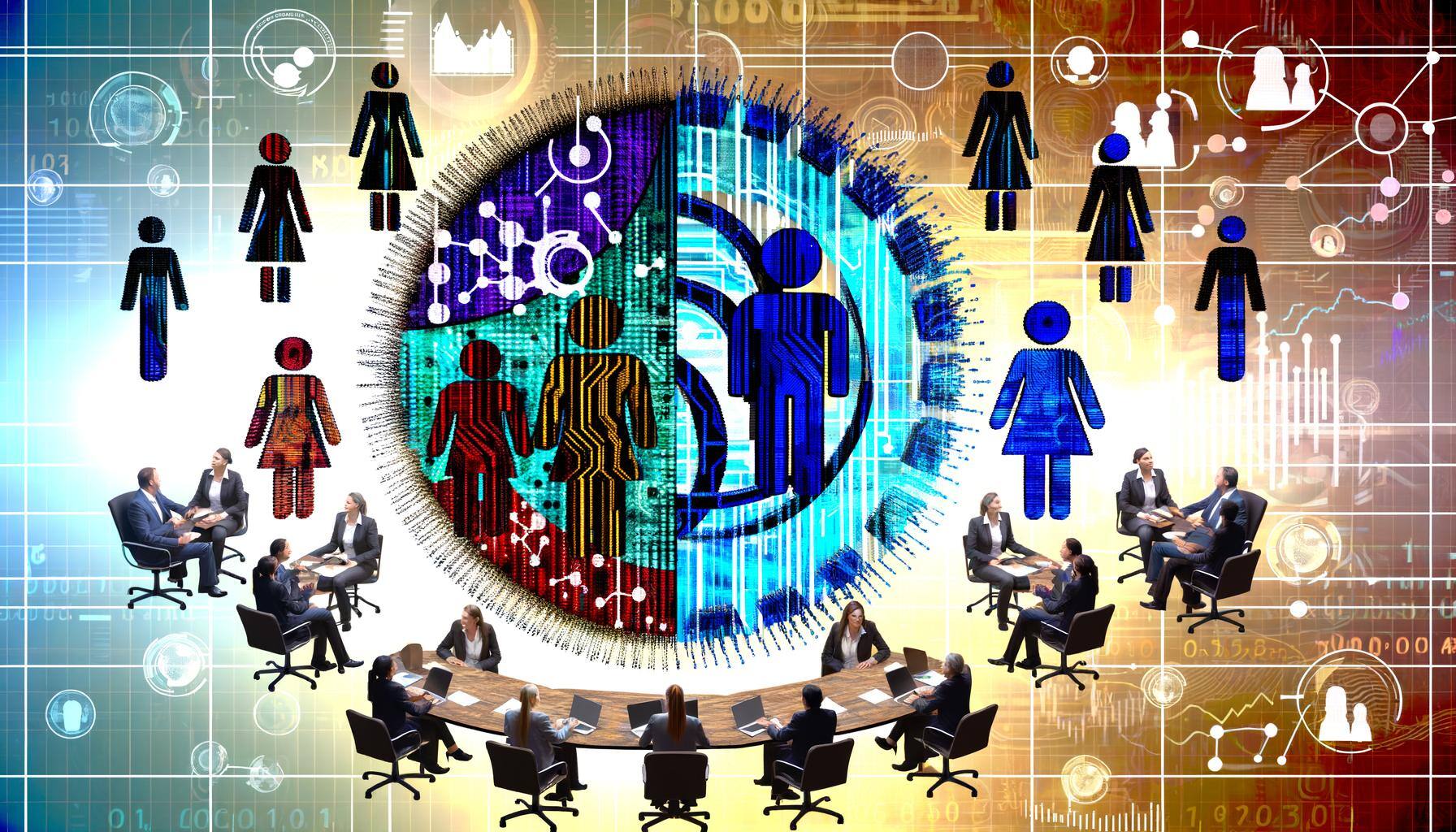
Responsible AI – Fairness
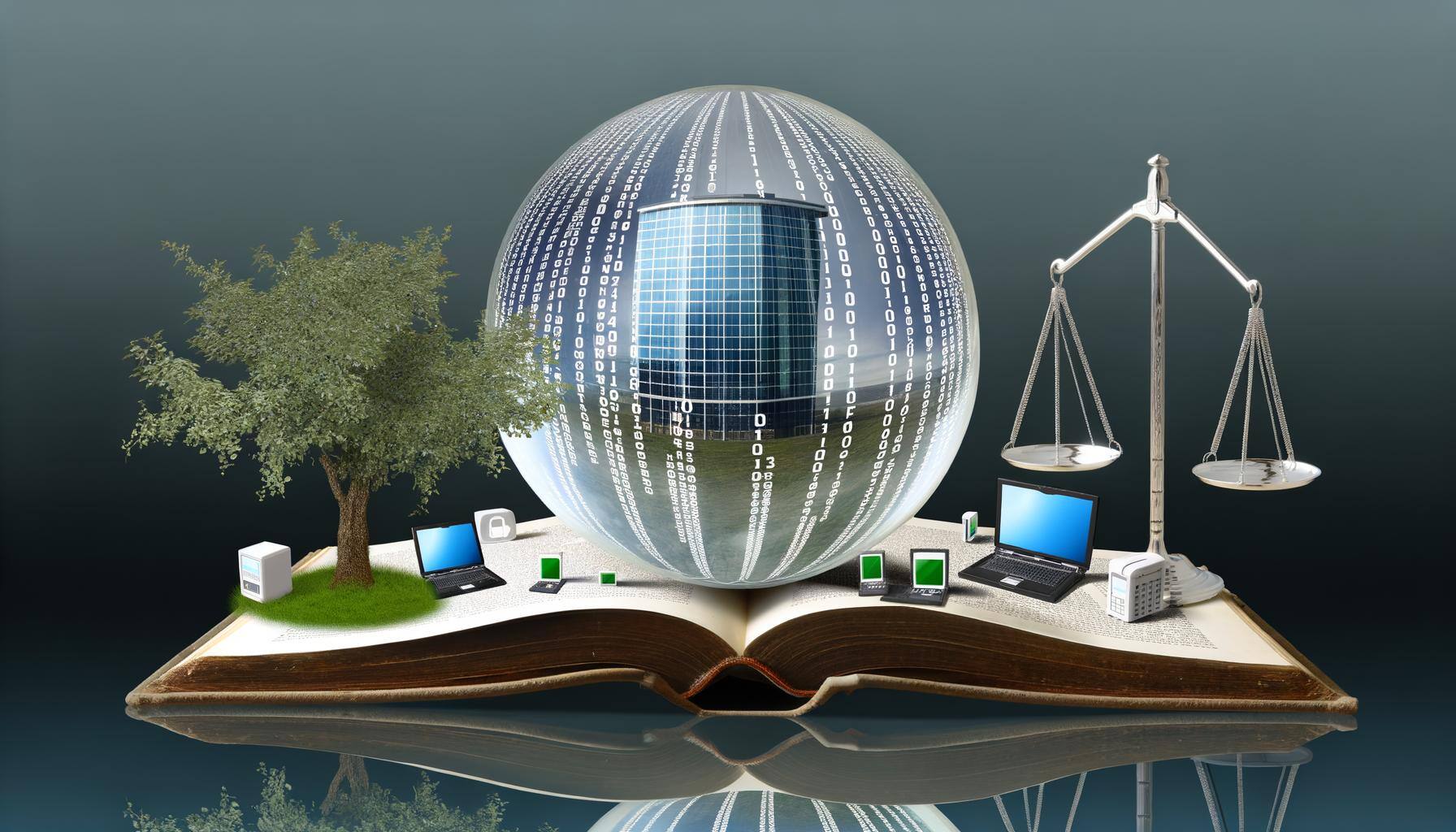
No Comments Yet
Let us know what you think