Responsible AI – Accountability
There are high-level “lenses” through which to develop, assess, and deploy products and services using AI, and these principles are components of a Responsible AI framework.
Fairness, Reliability & Safety, Privacy & Security, Inclusiveness, Transparency, and Accountability are the core principles we’ll be discussing in this series of blogs. In this blog we’re going to explore what Accountability means in terms of Responsible AI.
What is Accountability in a Responsible AI framework?
Accountability in Responsible AI involves ensuring that individuals and organizations responsible for designing, developing, and deploying AI systems are answerable for how these systems operate. It emphasizes that AI should not be the sole decision-maker in critical matters affecting individuals' lives and insists on maintaining human oversight. Establishing accountability involves setting industry standards and norms that guide the ethical deployment of AI technologies. This ensures that human values and ethical considerations steer AI operations, rather than letting the technology run without checks and balances.
Role of MLOps in Promoting Accountability
MLOps, or Machine Learning Operations, is a set of practices that aim to deploy and maintain machine learning models in production reliably and efficiently. MLOps can drive good accountability practices in several ways:
- Version Control and Audit Trails: MLOps ensures that all aspects of machine learning models, including data, code, and experiments, are versioned and tracked. This creates an audit trail that can be used to trace back through the model's lifecycle, identifying who made changes and when, which is crucial for accountability.
- Model Monitoring and Validation: Continuous monitoring of models in production ensures they perform as expected over time. MLOps facilitates the implementation of model validation steps and performance metrics that can trigger alerts if the model's behavior deviates from acceptable thresholds, prompting human intervention.
- Compliance and Reporting: MLOps frameworks can integrate compliance checks and reporting mechanisms that align with regulatory requirements and ethical standards, ensuring that the models adhere to necessary guidelines and that deviations are reported and addressed.
- Human-in-the-loop (HITL) Systems: MLOps can support the design and operation of HITL systems where human oversight is part of the AI decision-making process, ensuring that decisions can be reviewed and overridden by humans when needed.
How Accountability Informs Business Decisions
- Data-driven Insights: Through the causal inference components of Responsible AI dashboards, organizations can utilize historical data to understand the impact of specific actions or treatments. For example, analyzing how a new drug affects patients' blood pressure helps in making informed decisions about its use and distribution. These insights ensure that decisions are based on evidence and can be accounted for.
- Model-driven Insights: The counterfactual what-if analysis available in Responsible AI dashboards allows users to understand how different inputs or conditions might change an AI-driven decision. For instance, if a loan application is denied, the applicant can understand what factors might lead to a different decision in the future. This not only promotes transparency but also enhances accountability by allowing stakeholders to see the cause-and-effect relationship directly and adjust their actions accordingly.
By integrating accountability measures into the AI lifecycle through practices like MLOps and leveraging insights from Responsible AI tools, businesses can ensure that their AI systems are not only efficient and effective but also equitable, ethical, and aligned with broader societal values. This approach helps build trust among users and stakeholders, critical for the long-term success and integration of AI technologies in business processes.
Sector Examples of Accountability
Accountability can and should be considered when developing AI systems responsibly. Here are some examples illustrating how Accountability plays into Responsible AI initiatives:
- Healthcare
- Clinical Decision Support Systems: When developing AI systems that assist in diagnosing or recommending treatments, it's crucial to implement accountability by maintaining detailed logs of AI recommendations and corresponding clinician decisions. This ensures that there is a traceable path from AI suggestion to clinical action, allowing for accountability in cases where the AI's advice may lead to adverse outcomes.
- Regulatory Compliance: Healthcare entities can establish accountability by ensuring that all AI tools comply with HIPAA and other relevant regulations, protecting patient data and ensuring that systems are safe and reliable.
- Finance and Banking
- Credit Scoring and Loan Approvals: Banks and financial institutions can implement accountability by keeping comprehensive records of all AI model decisions and the factors influencing these decisions. In case of disputes or audits, these records can demonstrate compliance with fair lending laws and regulations.
- Fraud Detection Systems: Ensuring that there are mechanisms for review and appeal when AI systems flag transactions as fraudulent. This not only protects customers but also holds institutions accountable for the actions taken by their automated systems.
- Automotive and Transportation
- Autonomous Vehicles: Manufacturers can maintain accountability by developing detailed event recorders (similar to black boxes in airplanes) for autonomous vehicles. These recorders can log decisions made by the vehicle's AI systems, which can be crucial for liability purposes in the event of an accident.
- Traffic Management Systems: Cities and municipalities using AI to control traffic flow can ensure accountability by maintaining transparency about how data is collected and used, and by allowing public oversight and reporting on system performance and outcomes.
- Retail and E-commerce
- Personalized Recommendations: E-commerce platforms can implement accountability by transparently disclosing to users how their data is being used to generate personalized recommendations. Additionally, keeping logs that can audit the AI’s decision-making process helps address any biases or errors in the system.
- Inventory and Supply Chain Management: AI systems that automate inventory decisions can be made accountable by tracking decision rationales and outcomes, ensuring that decisions are explainable and justifiable.
- Human Resources
- Hiring and Recruitment Tools: Companies using AI-driven tools for screening and hiring must ensure these systems are accountable by regularly reviewing and auditing their performance to prevent discriminatory practices. Documentation of AI decision-making processes and criteria used must be accessible for compliance checks and fairness assessments.
- Employee Monitoring Systems: Accountability can be ensured by clearly communicating to employees what aspects of their performance or behavior are being monitored by AI systems and how these data influence decisions regarding their employment.
In each case, accountability in AI involves mechanisms for tracking and justifying decisions made by AI systems, providing clear guidelines and standards for operation, and maintaining human oversight where necessary. This not only helps in adhering to ethical standards but also builds trust with users and stakeholders affected by AI-driven decisions.
Summary
The challenge in Responsible AI is to navigate the application for principles to develop AI systems that are safe and reliable in a comprehensive sense. This often requires a multi-disciplinary approach that incorporates ethical, legal, technical, and social perspectives. Transparency, accountability, and ongoing engagement with stakeholders are also crucial to address these complexities effectively.
This is why having a framework, a well-rounded cross-disciplinary team involved, and a drive for an internal Center of Excellence is so important. It’s why we advise, support, engage, and champion the use of Responsible AI frameworks in organizations. If your organization would like to discuss this further, please contact us!
To read our Responsible AI White paper view here: https://infusedinnovations.com/responsible-ai
Stay connected. Join the Infused Innovations email list!
Share this
You May Also Like
These Related Posts
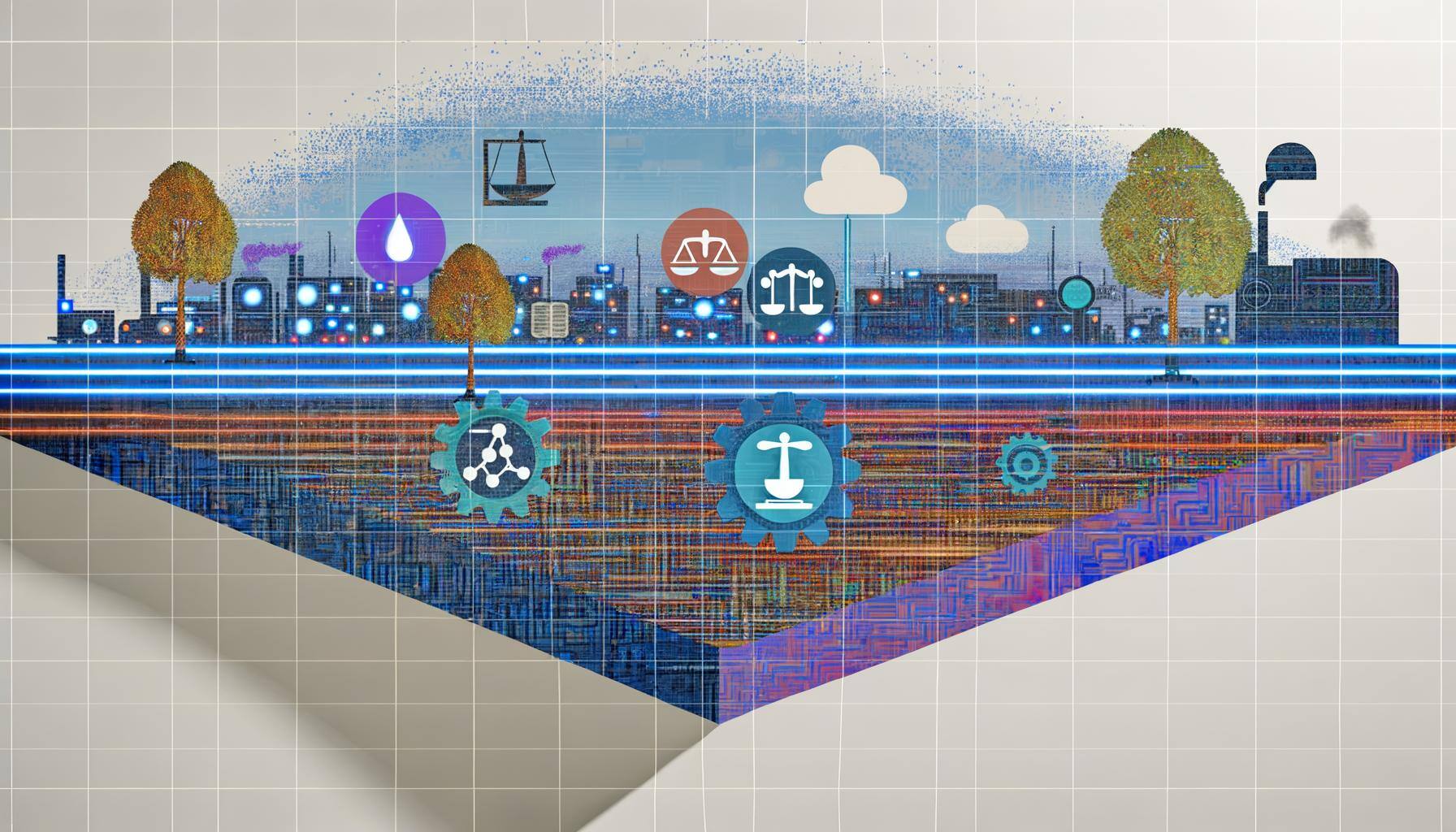
Responsible AI for Your Business
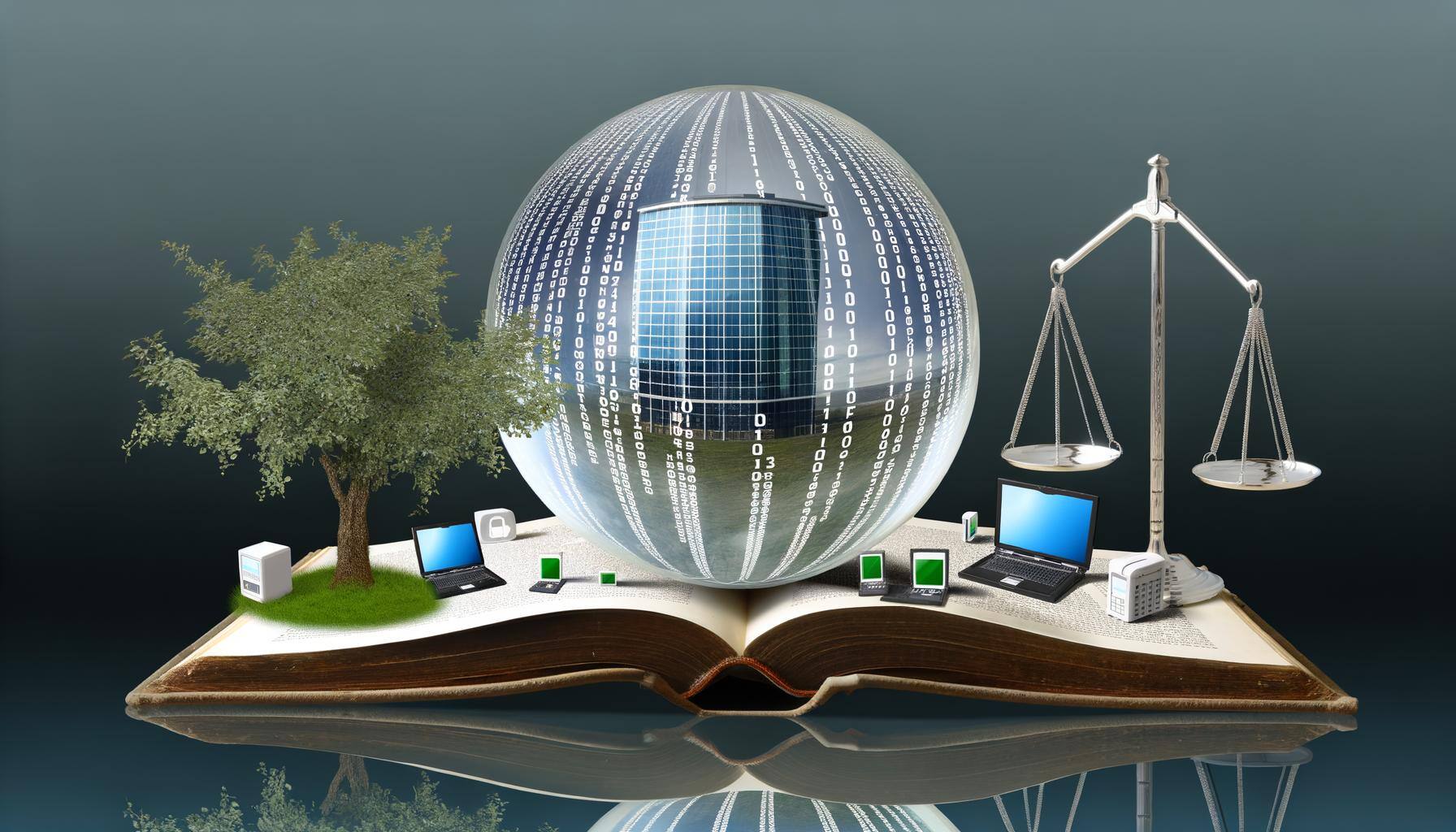
Responsible AI – Transparency
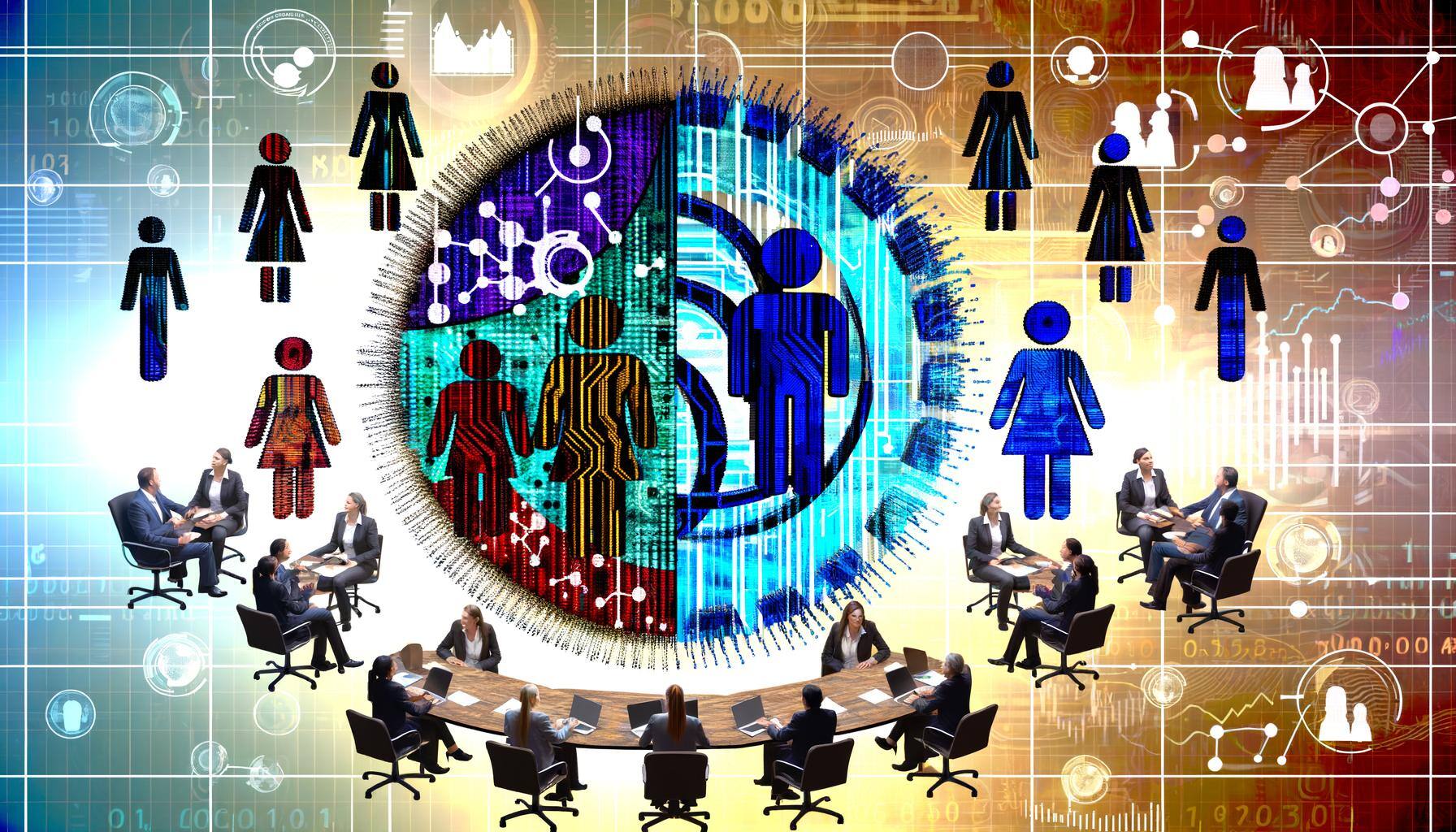
No Comments Yet
Let us know what you think