Responsible AI – Transparency
There are high-level “lenses” through which to develop, assess, and deploy products and services using AI, and these principles are components of a Responsible AI framework.
Fairness, Reliability & Safety, Privacy & Security, Inclusiveness, Transparency, and Accountability are the core principles we’ll be discussing in this series of blogs. In this blog we’re going to explore what Transparency means in terms of Responsible AI.
What is Transparency in a Responsible AI framework?
Transparency in Responsible AI refers to the clarity and openness with which AI systems operate and make decisions. This concept is vital when AI decisions have significant effects on people's lives, such as credit scoring or hiring processes. Transparency ensures that stakeholders, including users, developers, and those affected by AI decisions, can understand and trust the technology. A key aspect of transparency is interpretability, which involves providing clear, understandable explanations of how and why AI systems function and make decisions. This allows for the identification and rectification of issues related to performance, fairness, bias, and unintended outcomes.
Types of Interpretability in Transparency
- Global explanations: These provide an overview of the factors influencing the general behavior of an AI model. For instance, in a loan allocation model, global explanations would clarify which features (e.g., income level, credit score, employment history) are generally most influential in determining loan approvals.
- Local explanations: These focus on individual decisions made by the AI system. For example, explaining why a specific customer’s loan application was approved or rejected based on their unique data points, such as their specific income, existing debts, and credit history.
- Model explanations for a selected cohort of data points: This involves explaining the behavior of an AI model for specific groups or segments of data. For example, determining what features most significantly affect loan decisions for a subgroup like low-income applicants. This can help identify if the model behaves differently or unfairly for certain groups.
Examples of Transparency by Sector / Industry
- Finance and Banking: Transparency in AI systems used for credit scoring, loan approvals, and risk assessments helps customers and regulators understand the basis of financial decisions. For example, explaining why certain demographic groups might be receiving fewer loan approvals or higher interest rates.
- Healthcare: In medical diagnostics, AI systems that can explain their diagnostic decisions or treatment recommendations increase trust among patients and practitioners. Transparency is crucial for understanding AI-driven decisions in patient screening, treatment suggestions, or drug recommendations.
- Human Resources: AI applications in recruitment and hiring processes require transparency to ensure fairness and eliminate bias in candidate screening and selection. AI systems can be designed to explain why certain candidates were shortlisted or rejected based on their qualifications, experience, and other relevant attributes.
- Criminal Justice: Transparency in AI systems used for predictive policing, risk assessments, or parole decisions is critical to prevent bias against certain groups and ensure just outcomes. Explanations can help understand how the AI models predict the likelihood of reoffending or the suitability for parole for individuals.
- Retail and Marketing: AI-driven recommendation systems can benefit from transparency by explaining to customers why certain products are suggested, enhancing trust and user experience. This could involve disclosing the factors that lead to specific product recommendations, such as previous purchases, browsing history, or commonly bought items.
Summary
The challenge in Responsible AI is to navigate the application for principles to develop AI systems that are safe and reliable in a comprehensive sense. This often requires a multi-disciplinary approach that incorporates ethical, legal, technical, and social perspectives. Transparency, accountability, and ongoing engagement with stakeholders are also crucial to address these complexities effectively.
This is why having a framework, a well-rounded cross-disciplinary team involved, and a drive for an internal Center of Excellence is so important. It’s why we advise, support, engage, and champion the use of Responsible AI frameworks in organizations. If your organization would like to discuss this further, please contact us!
To read our Responsible AI White paper view here: https://infusedinnovations.com/responsible-ai
Stay connected. Join the Infused Innovations email list!
Share this
You May Also Like
These Related Posts
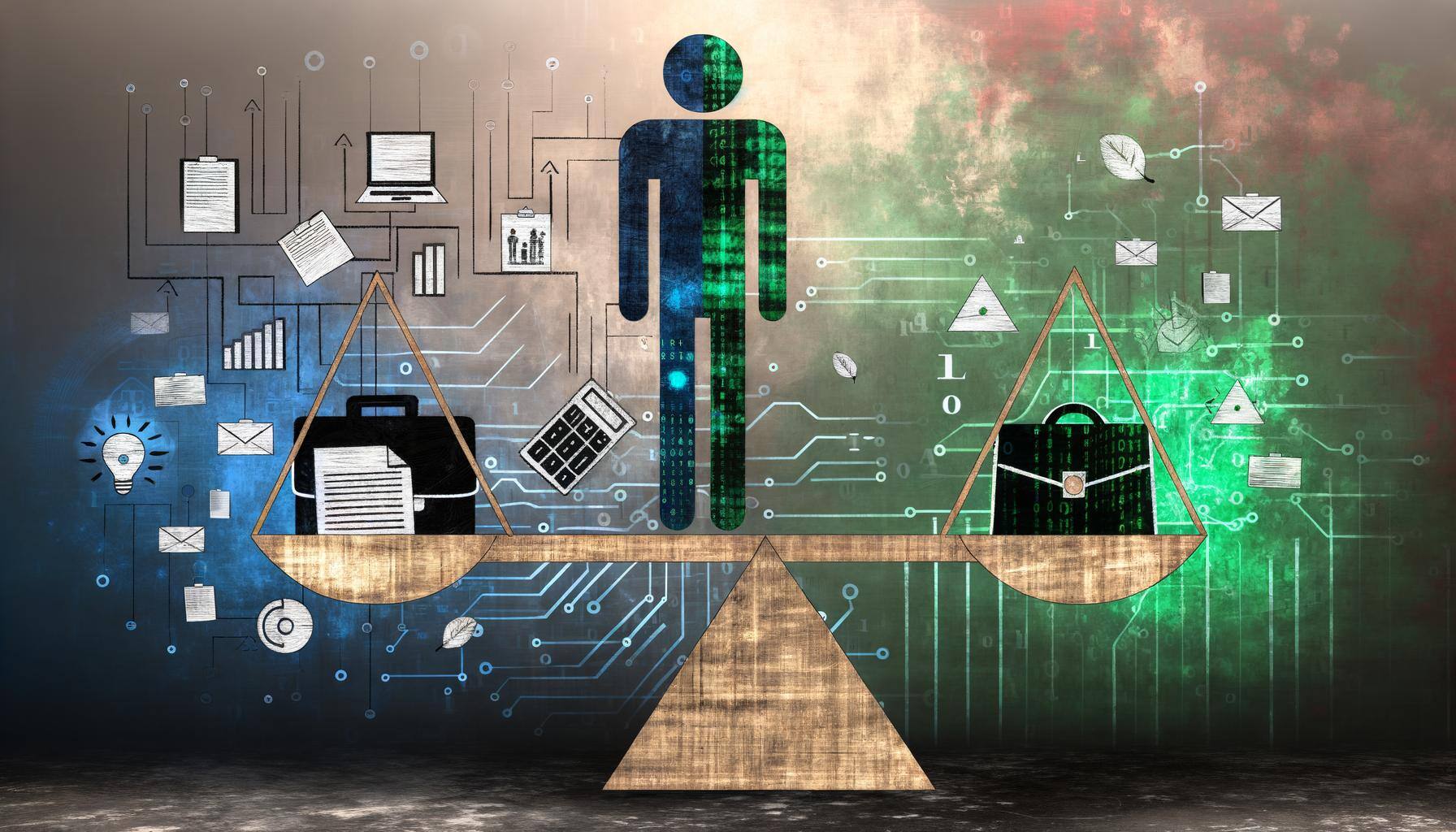
Responsible AI – Accountability
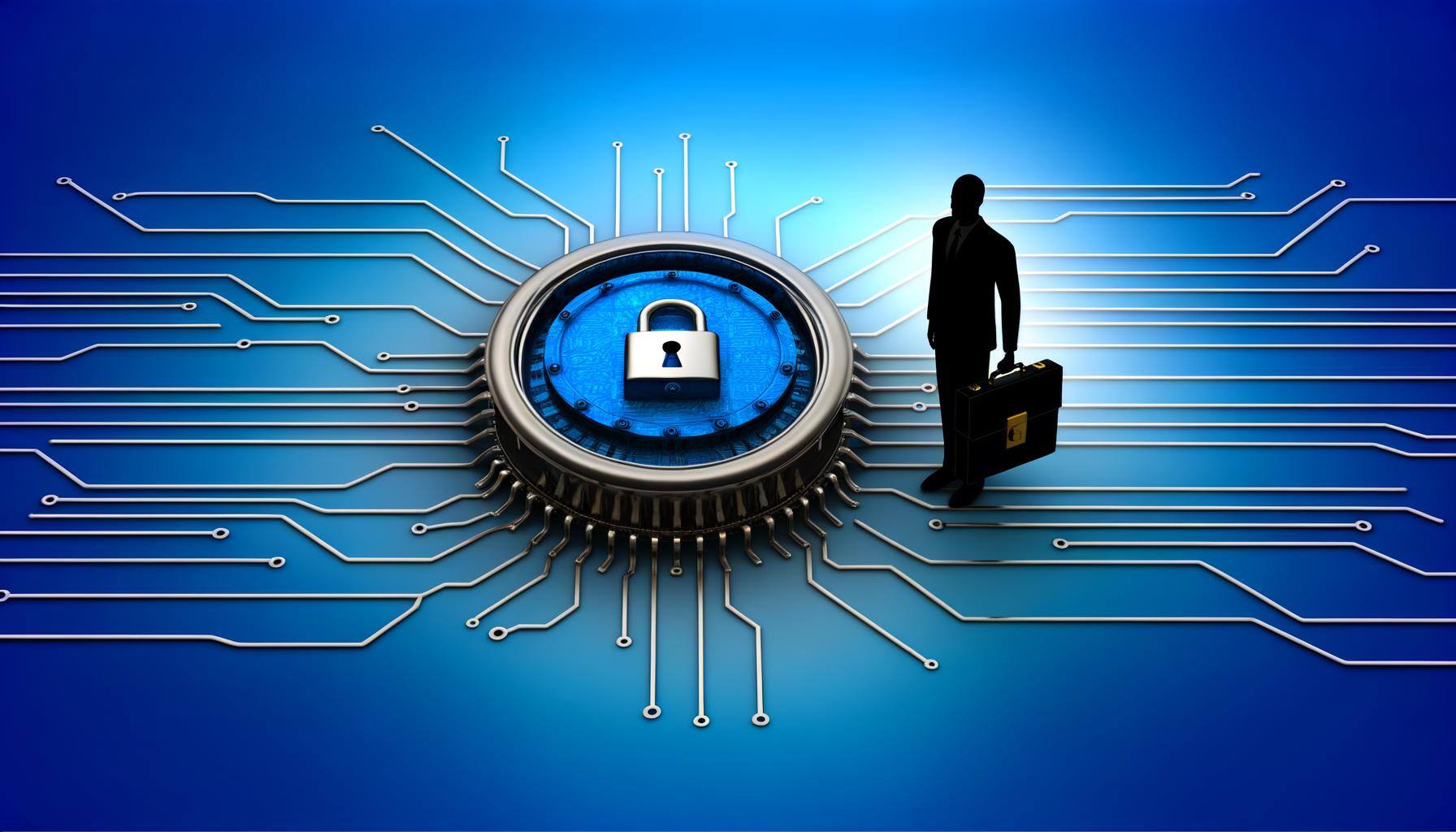
Responsible AI – Privacy & Security
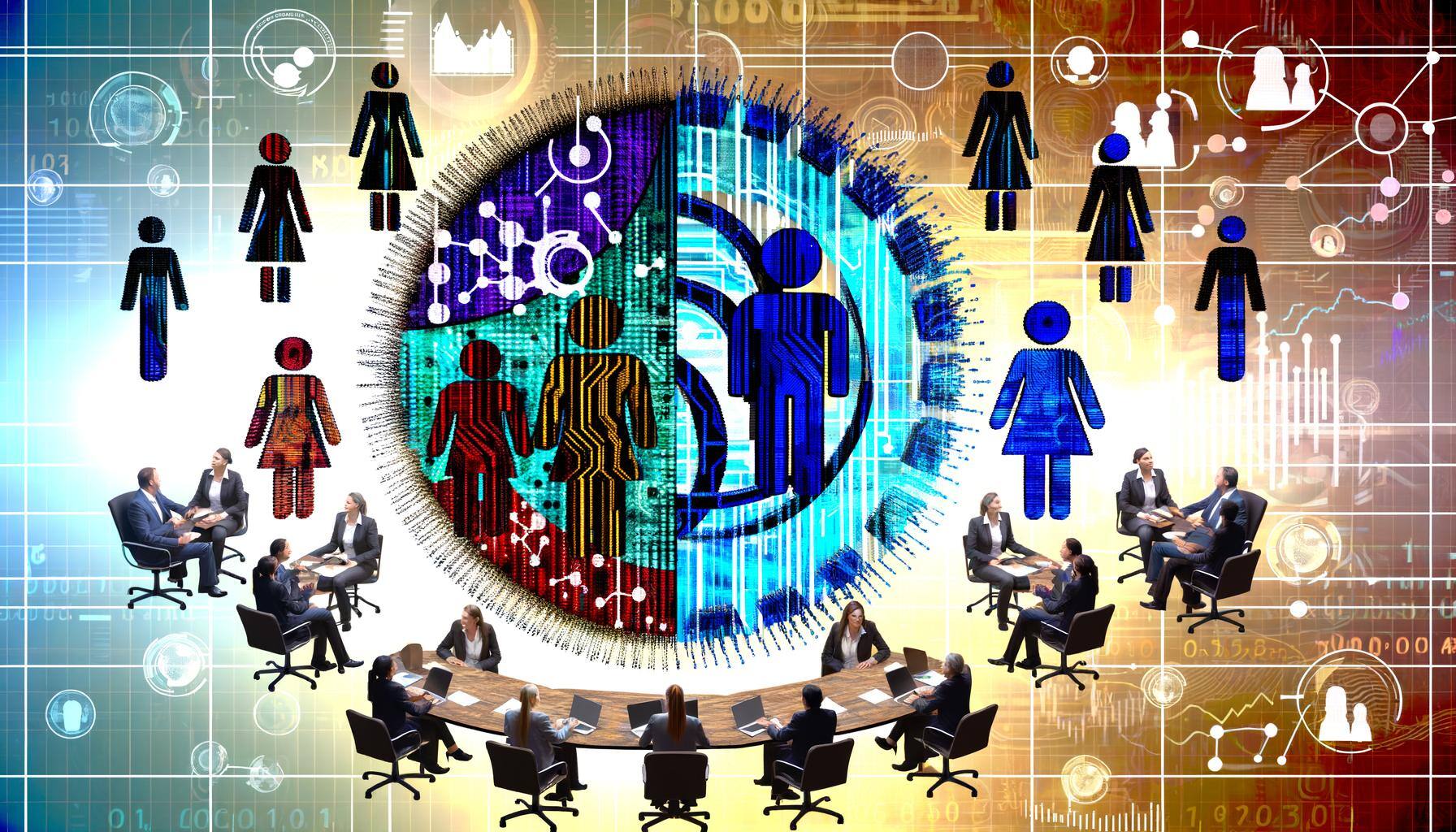
No Comments Yet
Let us know what you think