Responsible AI – Inclusiveness
There are high-level “lenses” through which to develop, assess, and deploy products and services using AI, and these principles are components of a Responsible AI framework.
Fairness, Reliability & Safety, Privacy & Security, Inclusiveness, Transparency, and Accountability are the core principles we’ll be discussing in this series of blogs. In this blog we’re going to explore what Inclusiveness means in terms of Responsible AI.
What is Inclusiveness in a Responsible AI framework?
Inclusiveness in the context of Responsible AI refers to the principle that AI systems should be designed and used in a way that they are accessible, usable, and beneficial to a diverse range of people, regardless of their age, gender, abilities, or ethnic backgrounds. This principle emphasizes the importance of engaging a broad spectrum of people in the development, deployment, and monitoring of AI systems to ensure that they do not perpetuate existing inequalities or create new forms of discrimination.
Types of Inclusiveness
Similar to our discussion previously around Fairness, inclusiveness in Responsible AI can also be viewed through different lenses, each addressing various dimensions of inclusivity. Understanding these distinct but interconnected aspects can help in designing and implementing AI systems that are truly inclusive. Here are a few key types to consider:
- Demographic Inclusiveness: This type focuses on ensuring that AI systems do not discriminate against individuals based on inherent characteristics such as age, race, gender, ethnicity, or disability. It involves designing AI technologies that are accessible to and usable by people with diverse traits, ensuring equitable benefits and opportunities.
- Socioeconomic Inclusiveness: AI should be accessible and beneficial not only to those in affluent or technologically advanced regions but also to those in underprivileged or under-resourced areas. This includes creating affordable AI solutions and ensuring they are deployable in low-resource settings, thus bridging the digital divide.
- Cultural and Linguistic Inclusiveness: AI systems should respect and understand cultural contexts and nuances. This type of inclusiveness ensures that AI interfaces, functionalities, and outputs are culturally sensitive and linguistically diverse, catering to users from different linguistic backgrounds and cultural practices.
- Cognitive and Physical Inclusiveness: This involves designing AI systems that are accessible to people with various physical and cognitive abilities. For example, voice-activated systems for those who cannot use traditional input devices, or simplified user interfaces for individuals with cognitive impairments. It aims to ensure that everyone can interact with AI technologies in a way that suits their individual capabilities.
- Geographical Inclusiveness: AI solutions should consider users in different geographical locations, including those in rural or remote areas. This type of inclusiveness ensures that AI-driven services, such as internet connectivity, healthcare diagnostics, and educational tools, are equally effective regardless of where individuals live.
- Temporal Inclusiveness: This aspect considers the long-term accessibility and usability of AI systems. It involves designing AI that remains inclusive as societal norms and technological landscapes evolve. Temporal inclusiveness ensures that AI systems can adapt to future needs and conditions, maintaining their relevance and utility over time.
- Organizational Inclusiveness: In a corporate or institutional context, this refers to the use of AI to promote inclusivity within the organization. This could mean AI tools that assist in diverse hiring practices, support various work styles and environments, or facilitate inclusive decision-making processes.
By addressing these different types of inclusiveness, developers and policymakers can create AI systems that are more equitable and beneficial for a wider segment of the population, thereby adhering to the broader goals of Responsible AI. Each type of inclusiveness tackles specific barriers that might prevent individuals or groups from fully benefiting from AI technologies, ensuring a more comprehensive approach to inclusivity.
Sector Examples of Inclusiveness
- Healthcare:
- Personalized Treatment: AI systems can be designed to consider the unique genetic backgrounds, lifestyles, and environments of diverse patient groups to provide personalized treatment plans that are more effective and inclusive.
- Language Accessibility: AI-driven health advisories or virtual assistants can be programmed to communicate in multiple languages and dialects, making crucial health information accessible to non-native speakers or marginalized communities.
- Education:
- Learning Assistants: AI can be used to create adaptive learning platforms that adjust the content based on the individual’s learning pace, style, and needs, helping students with disabilities or those who need additional support to keep up with their peers.
- Cultural Inclusivity: Educational content recommended by AI systems can include examples, histories, and perspectives from various cultures and backgrounds, promoting inclusivity and reducing cultural bias.
- Finance:
- Credit Scoring: AI systems in finance should be designed to fairly assess the creditworthiness of individuals from different socioeconomic backgrounds, avoiding biases based on zip code, ethnicity, or gender.
- Financial Literacy Tools: Develop AI-driven tools that cater to varying levels of financial literacy, helping underserved communities better understand and manage their finances.
- Human Resources:
- Recruitment Tools: AI used in recruitment should be programmed to ignore demographic-related information such as name, gender, age, and race to focus solely on the qualifications and the merit of the candidates, promoting a more diverse and inclusive workforce.
- Accessibility Features: Implement AI-powered tools that assist differently-abled employees in their work, enhancing their productivity and integration into the workforce.
- Public Services:
- Smart City Initiatives: AI-driven public transportation systems could be optimized to be more accessible for the elderly or people with disabilities, including features like voice-activated systems, wheelchair-friendly services, and visual aids for the visually impaired.
- Emergency Response: AI applications can be used to tailor emergency response services to the specific needs of diverse communities, ensuring that all citizens receive appropriate and timely aid during crises.
Summary
The challenge in Responsible AI is to navigate the application for principles to develop AI systems that are safe and reliable in a comprehensive sense. This often requires a multi-disciplinary approach that incorporates ethical, legal, technical, and social perspectives. Transparency, accountability, and ongoing engagement with stakeholders are also crucial to address these complexities effectively.
This is why having a framework, a well-rounded cross-disciplinary team involved, and a drive for an internal Center of Excellence is so important. It’s why we advise, support, engage, and champion the use of Responsible AI frameworks in organizations. If your organization would like to discuss this further, please contact us!
To read our Responsible AI White paper view here: https://infusedinnovations.com/responsible-ai
Stay connected. Join the Infused Innovations email list!
Share this
You May Also Like
These Related Posts
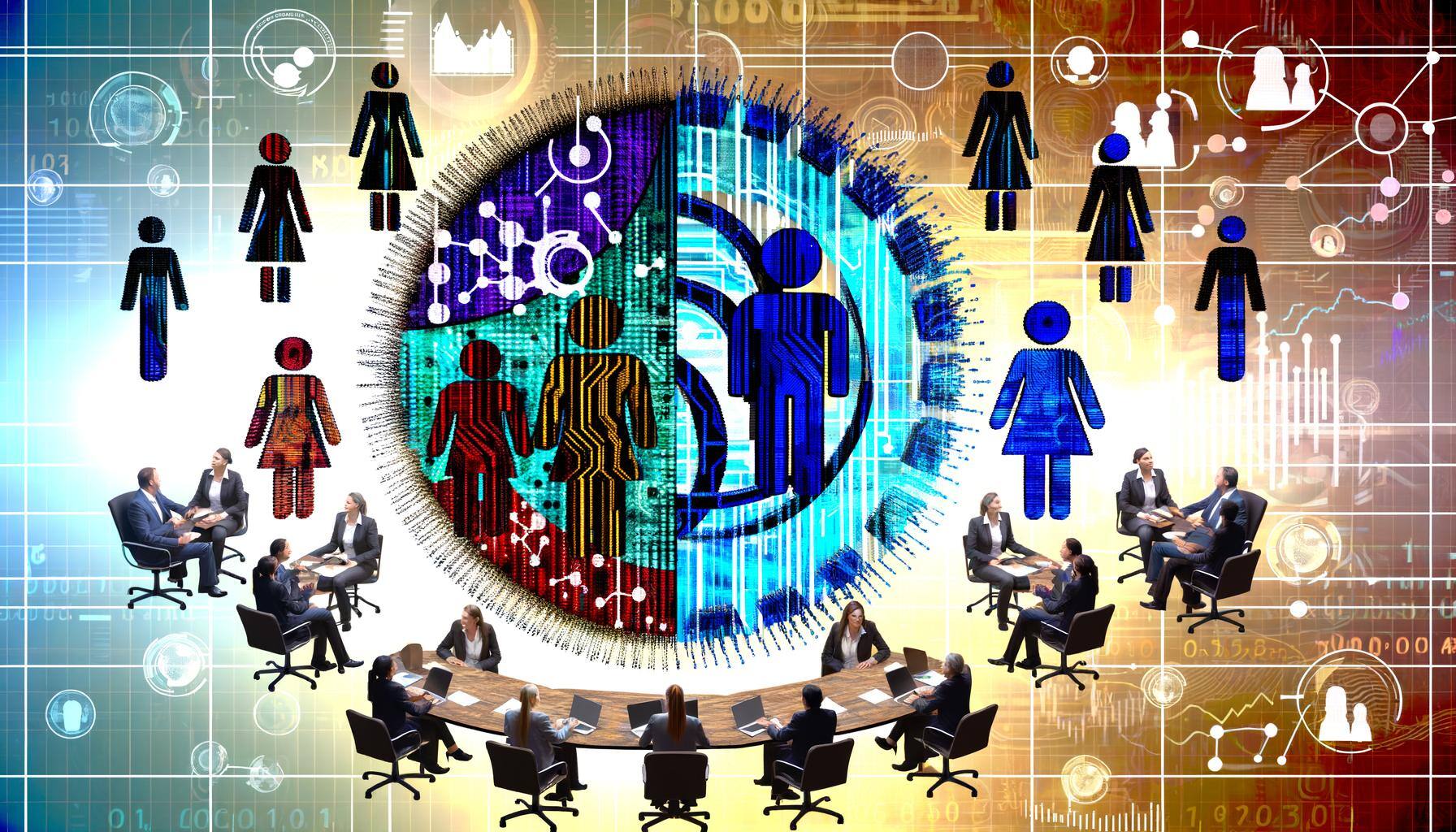
Responsible AI – Fairness
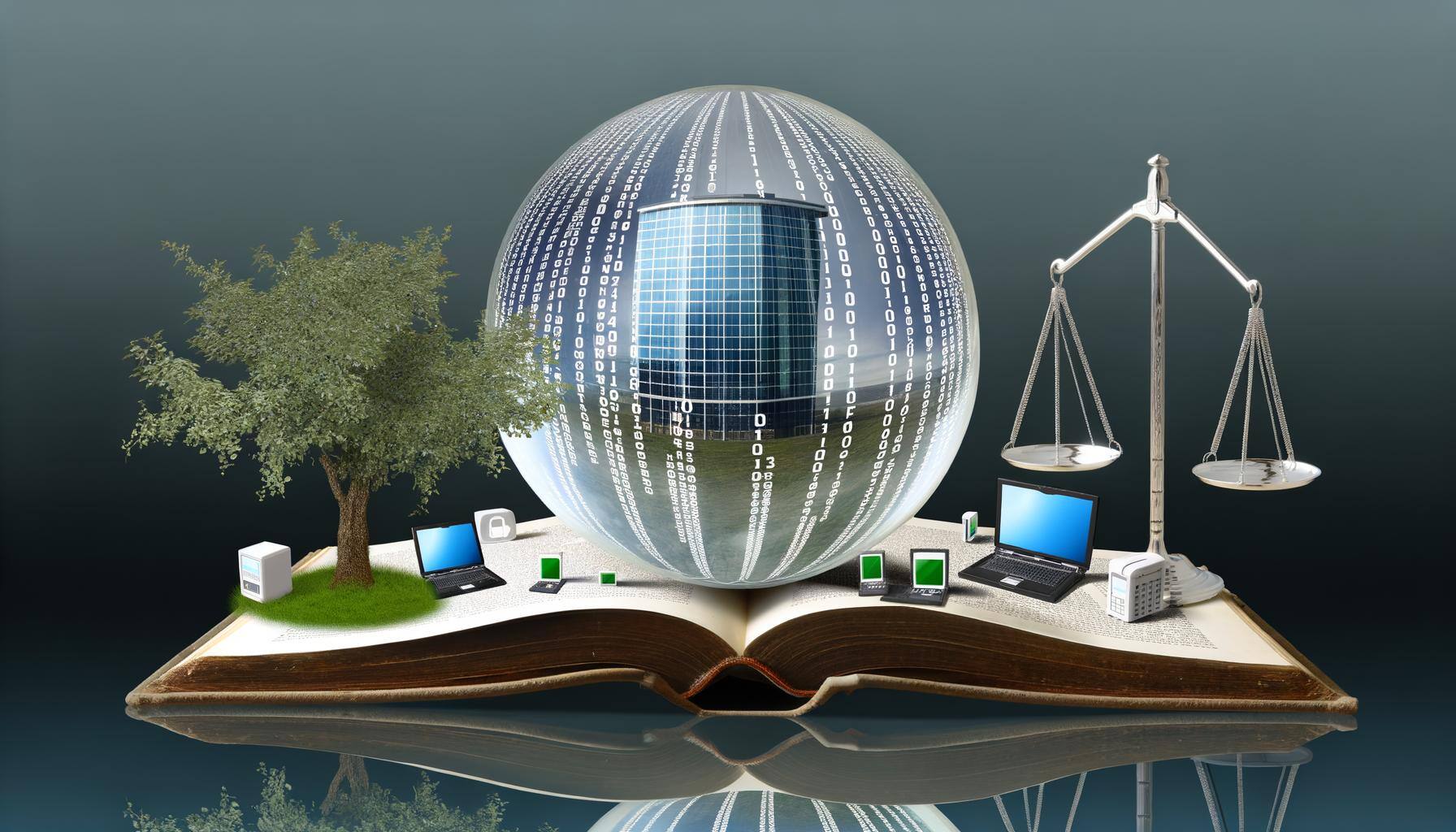
Responsible AI – Transparency
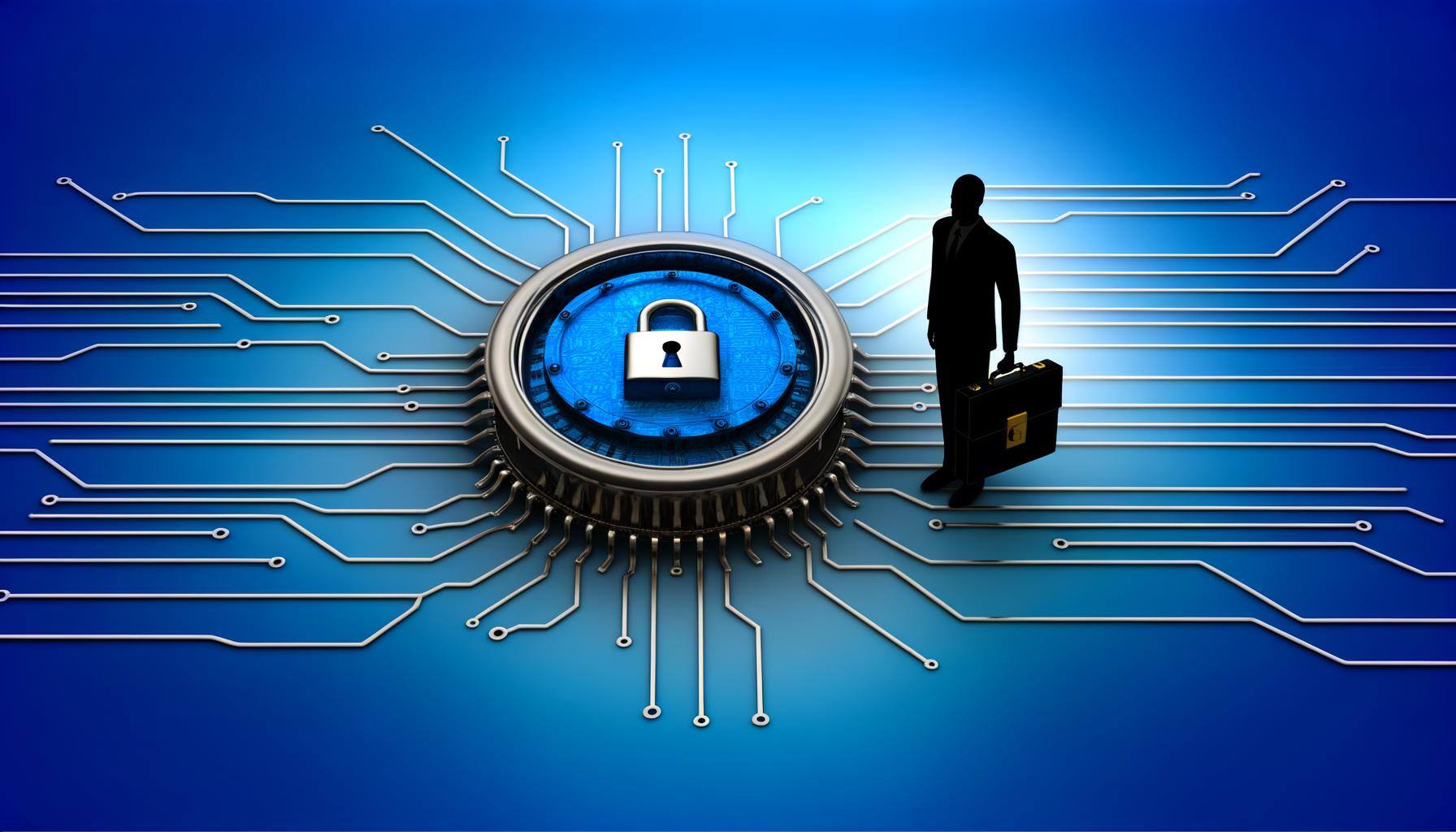
No Comments Yet
Let us know what you think