Responsible AI – Fairness
We’ve spoken with many prospects and customers about Responsible AI over the years. We always welcome (and encourage) debate in these conversations, not only because the usage of AI is ever-evolving, but because debating the meaning and applicability of various Responsible AI framework topics matters in the delivery of excellence.
While there are a number of different Responsible AI frameworks, there is significant overlap (as should be expected) between them in the high-level “lenses” through which to develop, assess, and deploy products and services.
Fairness, Reliability & Safety, Privacy & Security, Inclusiveness, Transparency, and Accountability are the core principles we’ll be discussing in this series of blogs, starting with Fairness.
Recently, while discussing the principle of Fairness in Responsible AI, we were asked to dive a bit deeper into the different types of Fairness.
What is Fairness in a Responsible AI framework?
At a high level, Fairness in the context of Responsible AI refers to the principle that AI systems should be designed and operated in such a way that they treat all individuals and groups equitably. This means ensuring that the AI does not perpetuate, exacerbate, or create biases based on race, gender, ethnicity, disability, age, sexual orientation, or other characteristics that are irrelevant to the decisions being made.
As the questioner noted, this is complicated. What if an insurance company wants to use AI to assess risk and calculate premiums? Using the data in a way that is fair to the company (since they are the ones that have to pay out in the event of some risk event happening and the calculated probabilities are what determine the premiums) is not the same as being fair societally (where we are understanding that in an insurance model we are spreading some risk around, there will be winners and losers).
Different Types of Fairness
Fairness can be defined and interpreted differently depending on the perspectives and values of different stakeholders. Let’s discuss Societal Fairness, Organizational Fairness, Technical Fairness, and User-Centric Fairness.
- Societal Fairness: From a societal perspective, fairness often involves broader considerations of equity, social justice, and the historical context of discrimination. This can include efforts to correct or compensate for existing inequalities. Society might focus on outcomes that ensure historically marginalized groups receive proportional benefits or opportunities.
- Organizational Fairness: Companies might focus on fairness as it relates to compliance with legal standards and avoiding litigation. Fairness within an organization might be more narrowly defined around specific business practices like hiring, lending, or advertising, ensuring that these processes are free from biases that violate regulations.
- Technical Fairness: From a technical standpoint, fairness is often about the statistical and mathematical treatment of data used by AI systems. It involves developing algorithms that do not create or perpetuate bias. This can be challenging, as it requires identifying what constitutes a bias and deciding on the fairness metrics to be used, such as demographic parity, equality of opportunity, or individual fairness.
- User-Centric Fairness: This perspective focuses on the fairness perceptions of the users or those directly affected by the AI system. It involves engaging with these stakeholders to understand their views and values concerning fairness, which can vary widely.
Real World Examples
There are several other examples and contexts where the conflict between different types of fairness in AI can be observed. Here are a few examples across various industries:
Credit Scoring:
- Societal Fairness: There's an expectation that financial services should be accessible to everyone, and that decisions around creditworthiness should not be biased by factors such as race, gender, or socioeconomic background.
- Organizational Fairness: Banks and lending institutions, however, need to manage risk effectively to remain profitable. Using AI to predict creditworthiness based on historical data might inadvertently incorporate past societal biases.
Employment and HR:
- Societal Fairness: Employment decisions should be based on merit and qualifications without discrimination against certain groups.
- Organizational Fairness: Companies may use AI for hiring to predict job performance based on historical data, which could reflect previous hiring biases or systemic inequalities in similar roles.
- Technical Fairness: Algorithms may not accurately assess individuals from underrepresented groups if the training data does not sufficiently include diverse examples.
Healthcare:
- Societal Fairness: AI in healthcare should aim for equitable treatment and outcomes for all patients, regardless of their background.
- Organizational Fairness: Healthcare providers might use AI to optimize resource allocation or treatment plans based on historical data, which could reflect and perpetuate existing health disparities.
- User-Centric Fairness: Patients expect personalized treatment that accurately reflects their individual health needs, which may conflict with generalized AI-driven decisions.
Criminal Justice:
- Societal Fairness: There is a critical need for fairness in legal systems, where decisions should not be influenced by race, gender, or other irrelevant factors.
- Organizational Fairness: Law enforcement agencies might use AI for predictive policing or to assess the risk of reoffending, but these systems can be biased if they rely on skewed data, such as arrest records that disproportionately represent certain groups.
Education:
- Societal Fairness: Educational tools and resources should be accessible and beneficial to all students, regardless of their background.
- Organizational Fairness: Educational institutions might use AI to streamline operations or personalize learning experiences, but these algorithms can perpetuate existing gaps in educational achievement if they are not carefully designed.
Each of these examples illustrates the challenge of balancing different interpretations of Fairness when deploying AI systems. It is crucial for developers, policymakers, and stakeholders to engage in continuous dialogue and ethical reflection to navigate these complex issues and strive for solutions that consider multiple perspectives on fairness.
Summary
The challenge in Responsible AI is to navigate these varying “definitions” of Fairness and to develop AI systems that are fair in a comprehensive sense. This often requires a multi-disciplinary approach that incorporates ethical, legal, technical, and social perspectives. Transparency, accountability, and ongoing engagement with stakeholders are also crucial to address these complexities effectively.
This is why having a framework, a well-rounded cross-disciplinary team involved, and a drive for an internal Center of Excellence is so important. It’s why we advise, support, engage, and champion the use of Responsible AI frameworks in organizations. If your organization would like to discuss this further, please contact us!
To read our Responsible AI White paper view here: https://infusedinnovations.com/responsible-ai
Stay connected. Join the Infused Innovations email list!
Share this
You May Also Like
These Related Posts
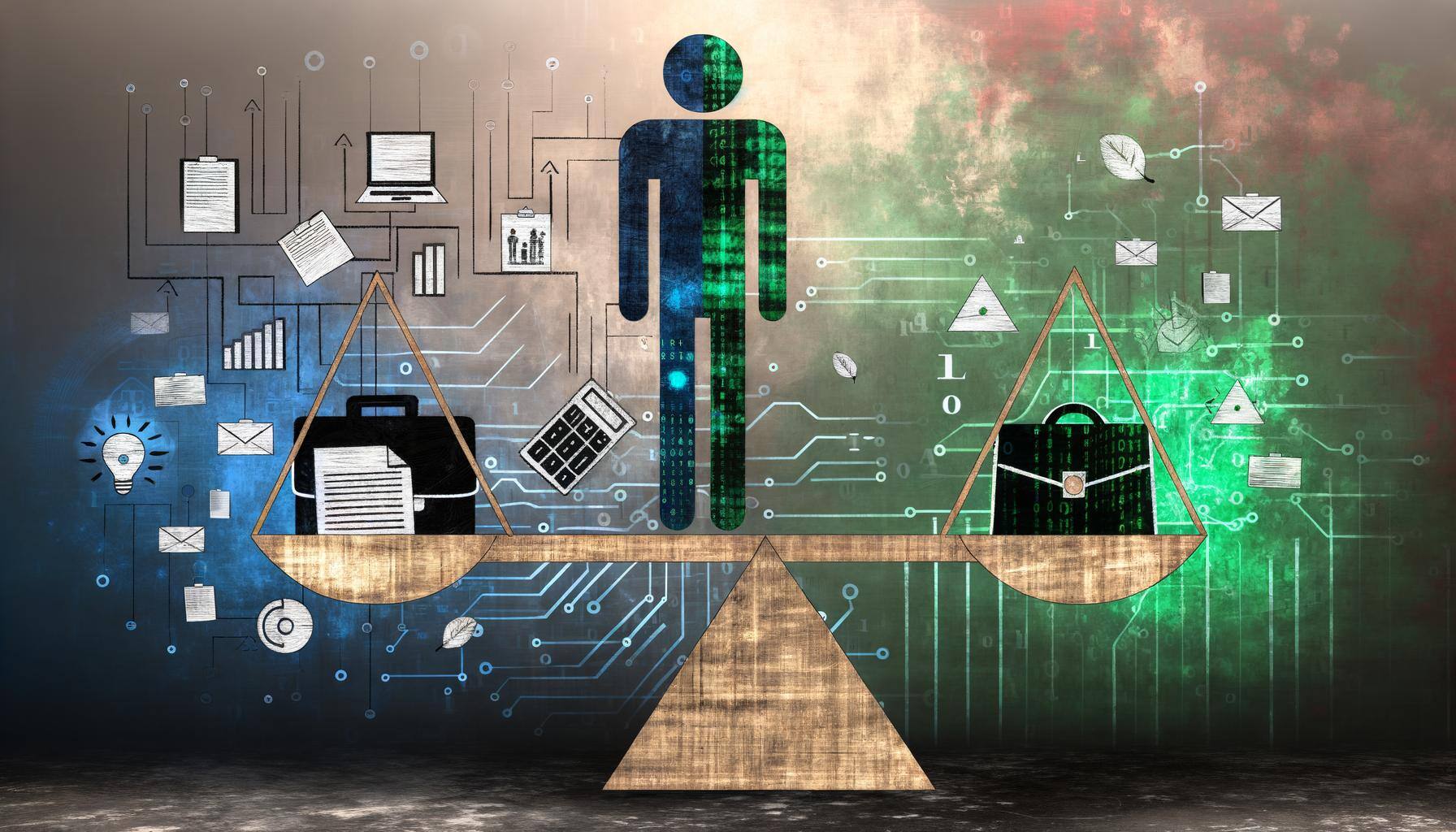
Responsible AI – Accountability
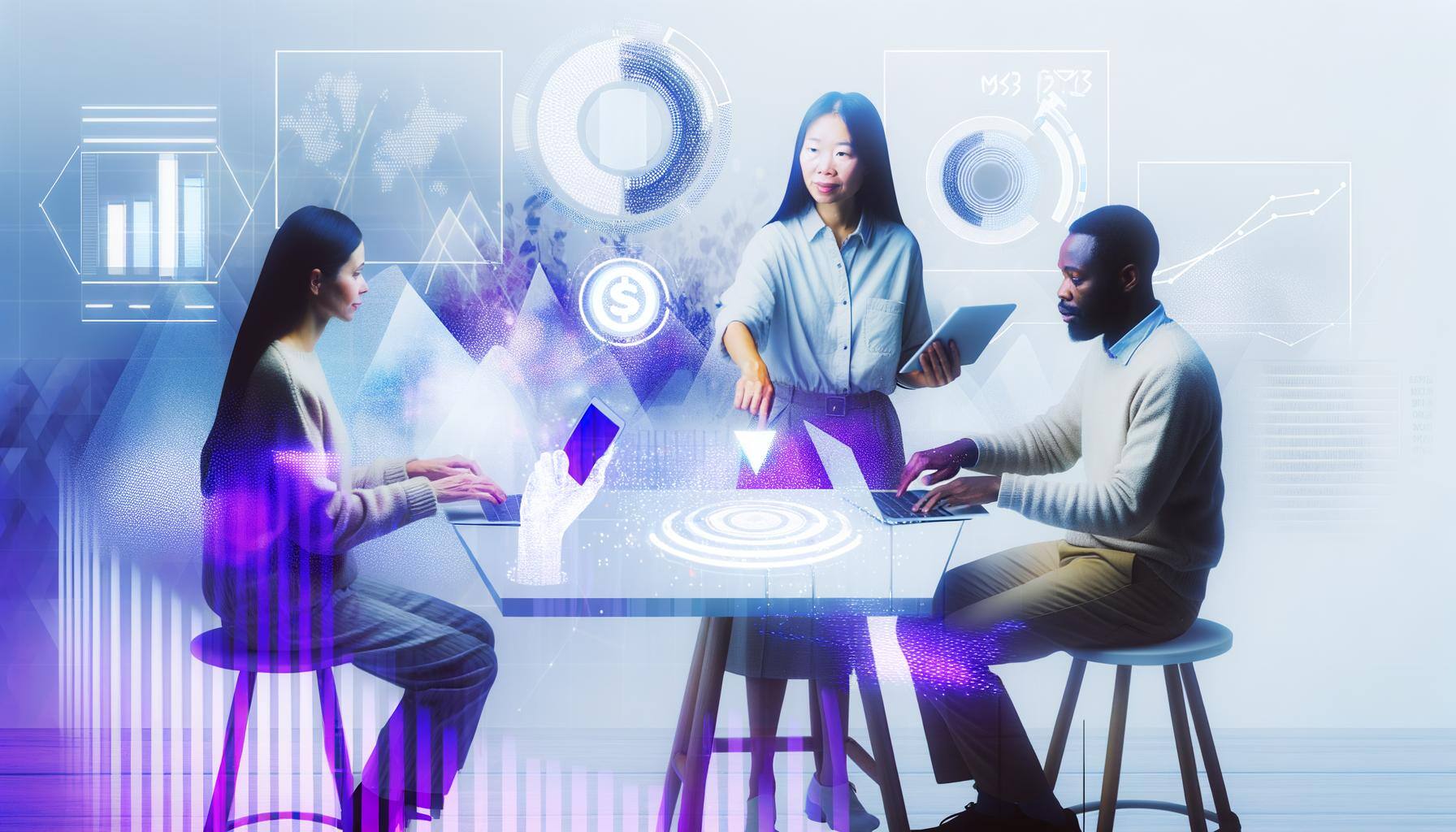
Responsible AI – Inclusiveness
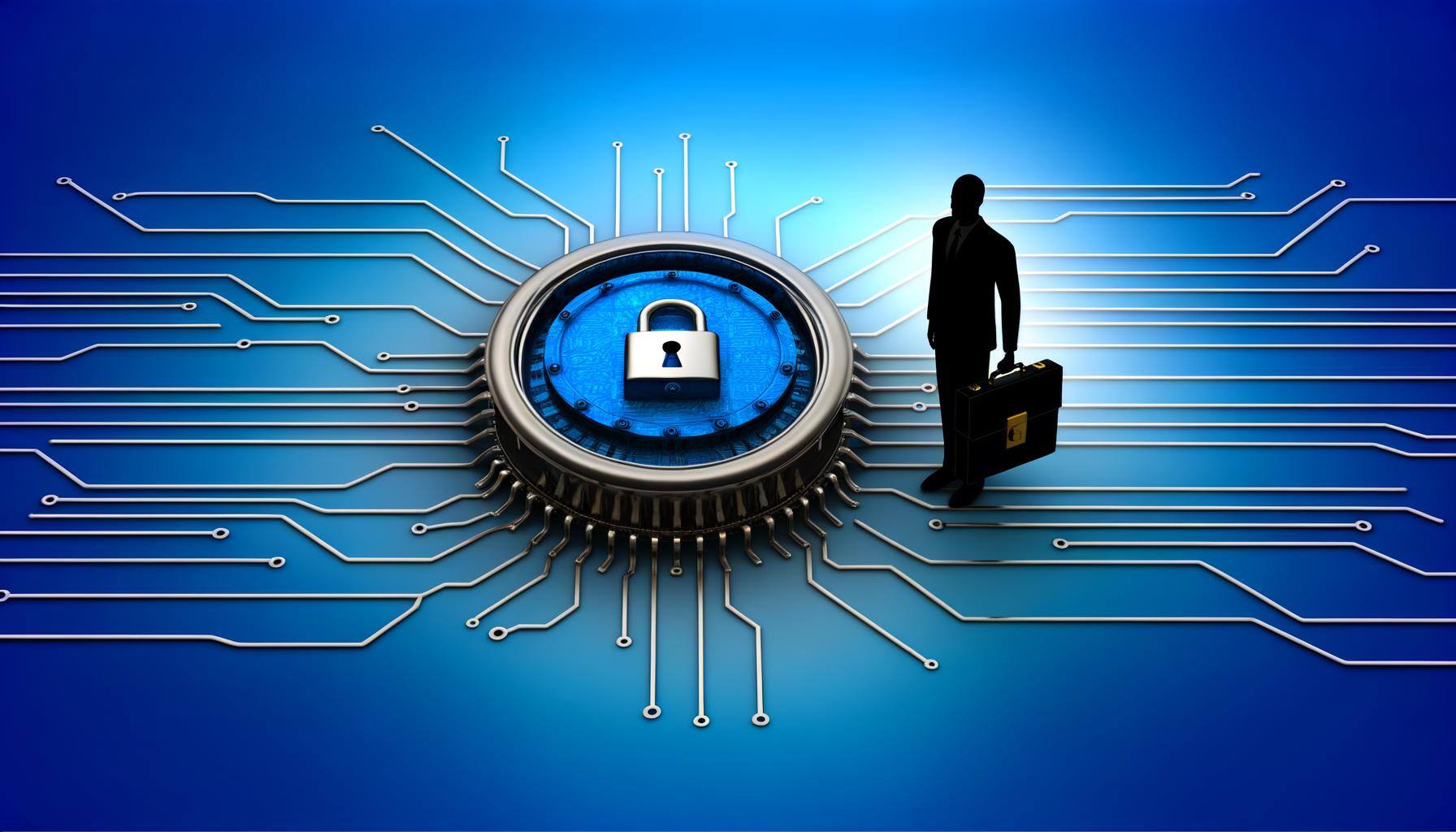
No Comments Yet
Let us know what you think