Responsible AI – Privacy & Security
As discussed in our previous blog posts on Fairness and Reliability & Safety in this series on Responsible AI principles, we’ve spoken with many prospects and customers about Responsible AI over the years and always welcome (and encourage) debate in these conversations.
Previously we had explained how there are high-level “lenses” through which to develop, assess, and deploy products and services using AI, and these principles are components of a Responsible AI framework.
Fairness, Reliability & Safety, Privacy & Security, Inclusiveness, Transparency, and Accountability are the core principles we’ll be discussing in this series of blogs. Our first blog explored Fairness in more depth, the second focused on Reliability & Safety. In this blog we are going to explore Privacy & Security in more detail.
What is Privacy & Security in a Responsible AI framework?
As AI becomes more prevalent, protecting privacy and securing personal and business information are becoming more important and complex. With AI, privacy and data security require close attention because access to data is essential for AI systems to make accurate and informed predictions and decisions about people. AI systems must comply with privacy laws that require transparency about the collection, use, and storage of data, and mandate that consumers have appropriate controls to choose how their data is used. It is important that administrators and developers create policies and configurations that:
- Restrict access to resources and operations by user account or group
- Restrict incoming and outgoing network communications
- Encrypt data in transit and at rest
- Scan for vulnerabilities
- Apply and audit configuration policies
The principles of Privacy & Security in a Responsible AI framework are essential to ensure that AI systems are designed, developed, and deployed in a manner that respects user privacy and maintains data security. A deeper five into this important principle requires consideration of a number of different areas:
Data Protection and Privacy:
- Privacy by Design: Incorporate privacy controls into the technology, at the design stage itself rather than as an afterthought.
- Data Minimization: Only collect data that is necessary for the specific purpose for which consent was given.
- Transparency: Clearly inform users about what data is being collected, how it will be used, and who will have access to it.
Security of AI Systems:
- Robustness: AI systems should be secure and resilient against attacks and failures. They should be able to detect and respond to security breaches and errors.
- Secure Data Storage and Transfer: Implement strong encryption methods for data at rest and in transit.
- Regular Audits and Updates: Continuously test and update AI systems to guard against potential vulnerabilities.
Access Controls:
- Authentication and Authorization: Ensure that only authorized individuals have access to sensitive data and AI systems.
- User Consent: Obtain explicit consent from users before collecting, using, or sharing their data.
Anonymization:
- De-identification: Remove personally identifiable information when storing or processing data to protect user privacy.
- Use of Synthetic Data: Employ synthetic data where possible to avoid using real user data in training AI models.
Accountability:
- Clear Responsibility: Assign clear responsibilities for privacy and security to specific roles within the organization.
- Audit Trails: Maintain logs of data access and processing activities to track misuse and ensure accountability.
Ethical Data Use:
- Fairness and Non-discrimination: Ensure the AI system does not use data in a way that discriminates against any individual or group.
- Purpose Limitation: Use data only for the specific purposes for which it was collected.
Compliance with Laws and Regulations:
- Legal Compliance: Adhere to all applicable laws, regulations, and standards related to privacy and data protection, such as GDPR (General Data Protection Regulation), HIPAA (Health Insurance Portability and Accountability Act), and others depending on the geographical location and sector.
Implementing these principles requires a multidisciplinary approach involving legal, ethical, technical, and operational expertise. Organizations should also engage with stakeholders, including customers, employees, and regulators, to ensure that their AI systems align with societal values and expectations regarding privacy and security.
Real World Examples
The principles of privacy and security are applied differently across various industries and use cases, tailored to address specific risks and regulatory requirements. Here are examples from a few industries:
Healthcare:
- Data Protection and Privacy: Healthcare data is highly sensitive. Under regulations like HIPAA in the U.S., patient data must be handled with strict privacy controls. For instance, when AI is used to predict patient outcomes based on electronic health records (EHRs), only de-identified data should be used unless explicit consent is obtained.
- Security of AI Systems: Healthcare providers implement robust encryption and access controls to protect against data breaches that could compromise patient privacy.
- Compliance with Laws and Regulations: AI applications in healthcare must comply with local and international regulations, ensuring that patient data is not misused or mishandled.
Finance:
- Anonymization: Financial institutions often anonymize transaction data before using it to train AI models for fraud detection. This protects customer privacy while allowing the detection systems to learn patterns of fraudulent behavior.
- Access Controls: Banks use sophisticated authentication mechanisms (e.g., biometrics, two-factor authentication) to restrict access to financial data and AI systems.
- Regular Audits and Updates: AI systems used for credit scoring or algorithmic trading are regularly audited to ensure they are secure and not being manipulated.
Retail:
- Data Minimization: When using AI for personalized marketing, retailers collect only the data necessary to enhance user experience, such as purchasing history and browsing behavior, while avoiding sensitive information unless absolutely necessary.
- User Consent: Retailers must obtain clear consent from users before collecting their data for AI-driven recommendations. Transparency about what data is collected and how it’s used is crucial.
- Ethical Data Use: Ensuring that AI systems do not use customer data to discriminate or unfairly target certain groups of users.
Automotive / Autonomous Vehicles:
- Security of AI Systems: Autonomous vehicles use AI to process vast amounts of data from sensors and cameras. Ensuring the integrity and security of this data is critical to prevent malicious attacks that could lead to safety hazards.
- Robustness: AI systems in autonomous vehicles are designed to be extremely robust against failures, with layers of redundancy to ensure safety even if one component fails.
Telecommunications:
- Secure Data Storage and Transfer: Telecom companies handle massive amounts of personal data, and in many cases use this data to know more about their customers and target advertising or other services to them. Using strong encryption for data at rest and in transit is essential to protect user privacy.
- Compliance with Laws and Regulations: Compliance with regulations such as GDPR is critical, especially for telecoms operating across multiple countries, necessitating rigorous data protection measures.
Education:
- User Consent: In educational apps and platforms utilizing AI, it’s important to obtain consent from users (or guardians, in the case of minors) before collecting data about learning patterns or performance.
- Anonymization: De-identifying data used to train AI models that personalize learning experiences or track student progress, to prevent any potential misuse of personal information.
In each of these cases, the implementation of Privacy & Security principles ensures that AI technologies enhance user benefits without compromising ethical standards or personal privacy. Regular updates, audits, and adherence to legal standards are common threads that run across all industries to maintain trust and security.
Summary
The challenge in Responsible AI is to navigate the application for principles to develop AI systems that are safe and reliable in a comprehensive sense. This often requires a multi-disciplinary approach that incorporates ethical, legal, technical, and social perspectives. Transparency, accountability, and ongoing engagement with stakeholders are also crucial to address these complexities effectively.
This is why having a framework, a well-rounded cross-disciplinary team involved, and a drive for an internal Center of Excellence is so important. It’s why we advise, support, engage, and champion the use of Responsible AI frameworks in organizations. If your organization would like to discuss this further, please contact us!
To read our Responsible AI White paper view here: https://infusedinnovations.com/responsible-ai
Stay connected. Join the Infused Innovations email list!
Share this
You May Also Like
These Related Posts
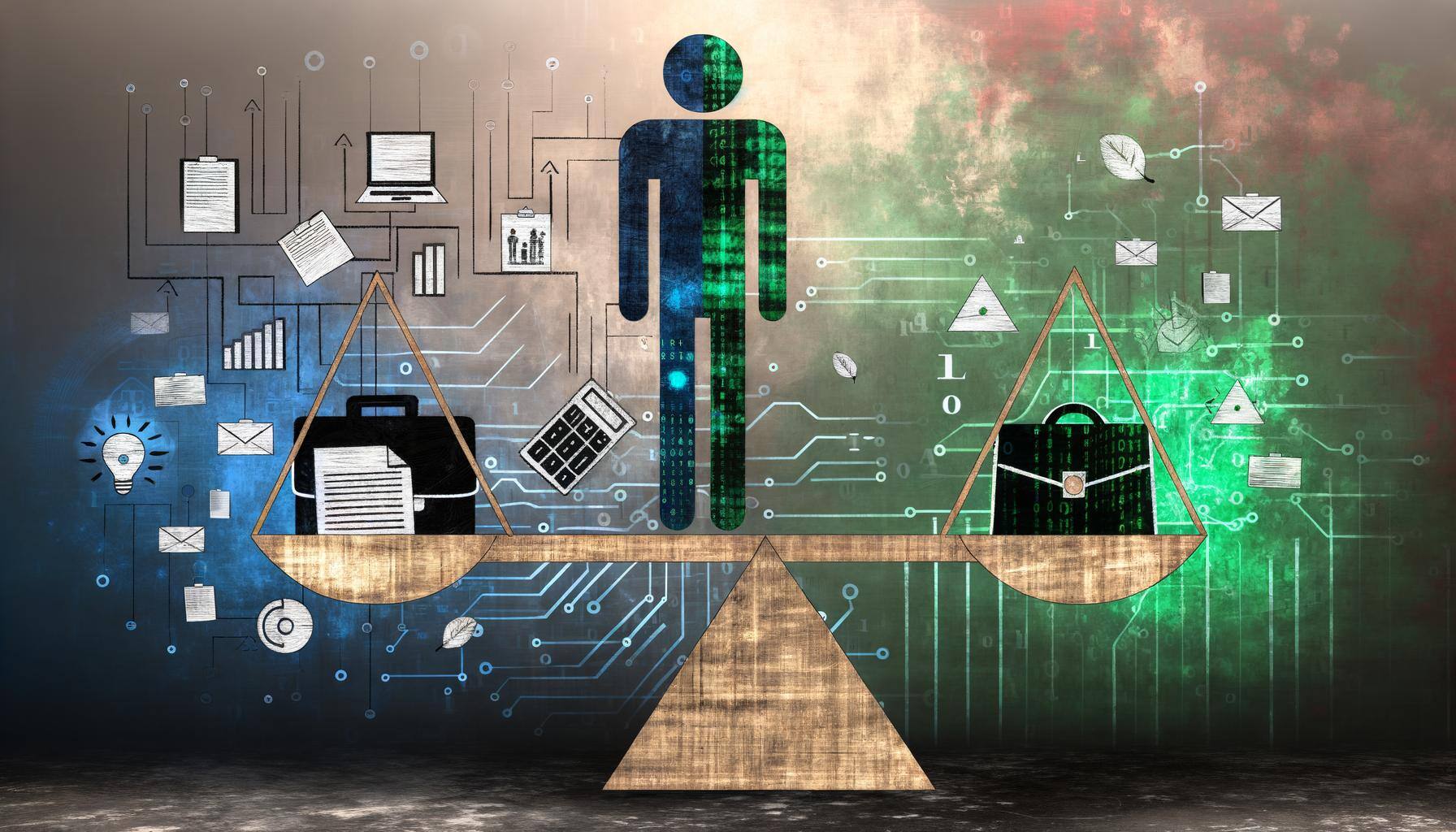
Responsible AI – Accountability
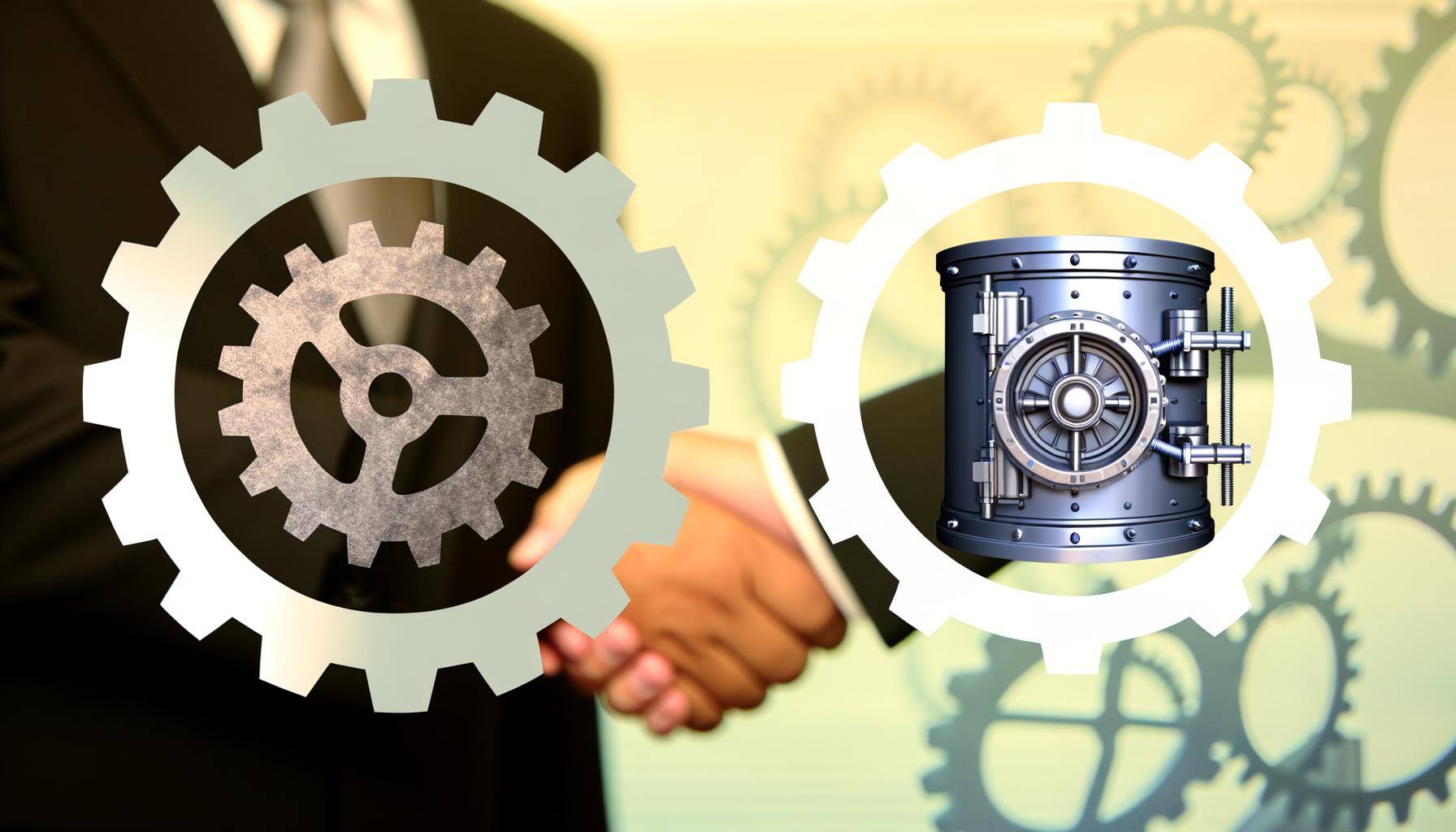
Responsible AI – Reliability & Safety
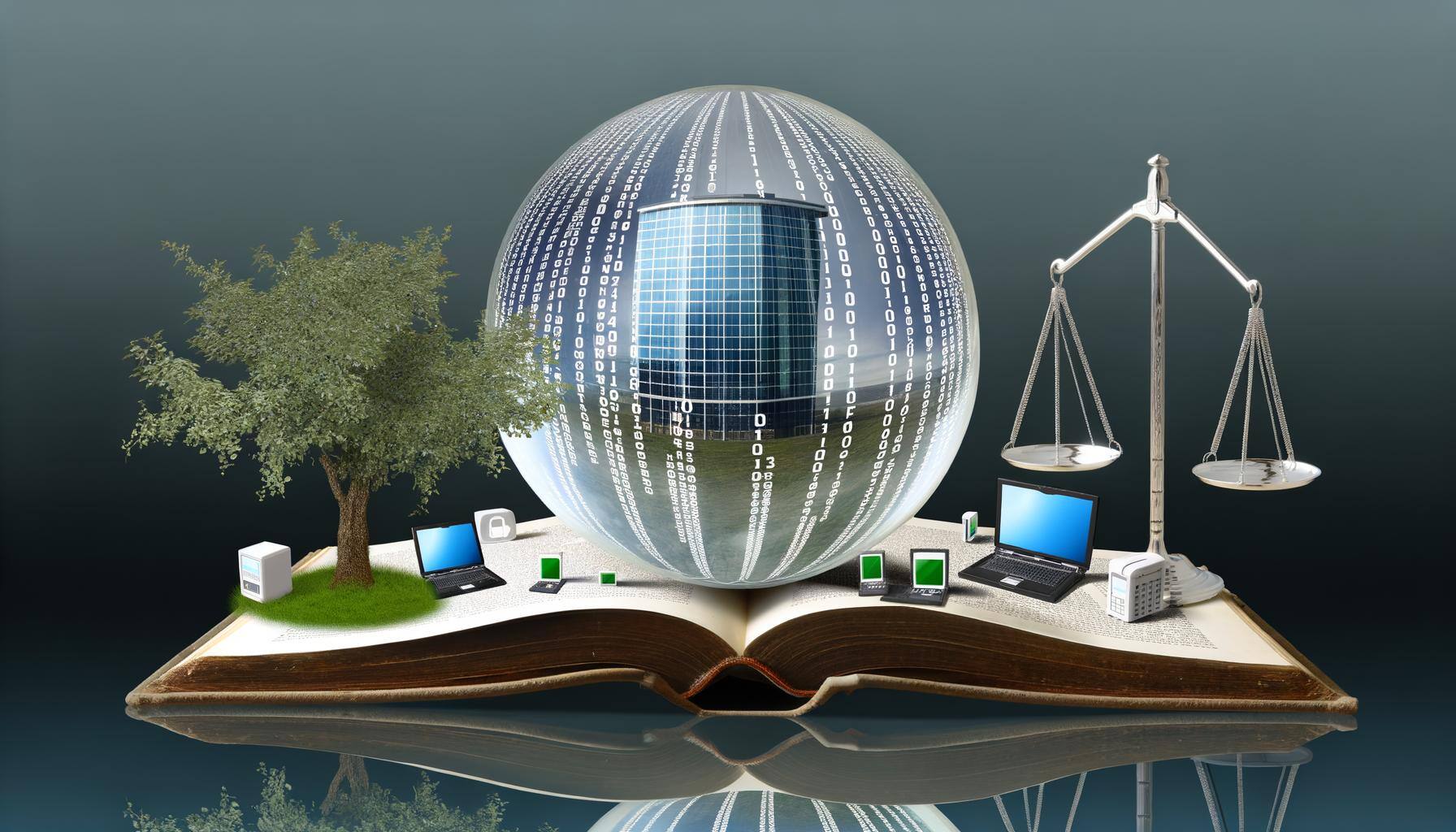
No Comments Yet
Let us know what you think