Using Machine Learning for Music Therapy
AI-Driven Music Generation for Mental Well-Being
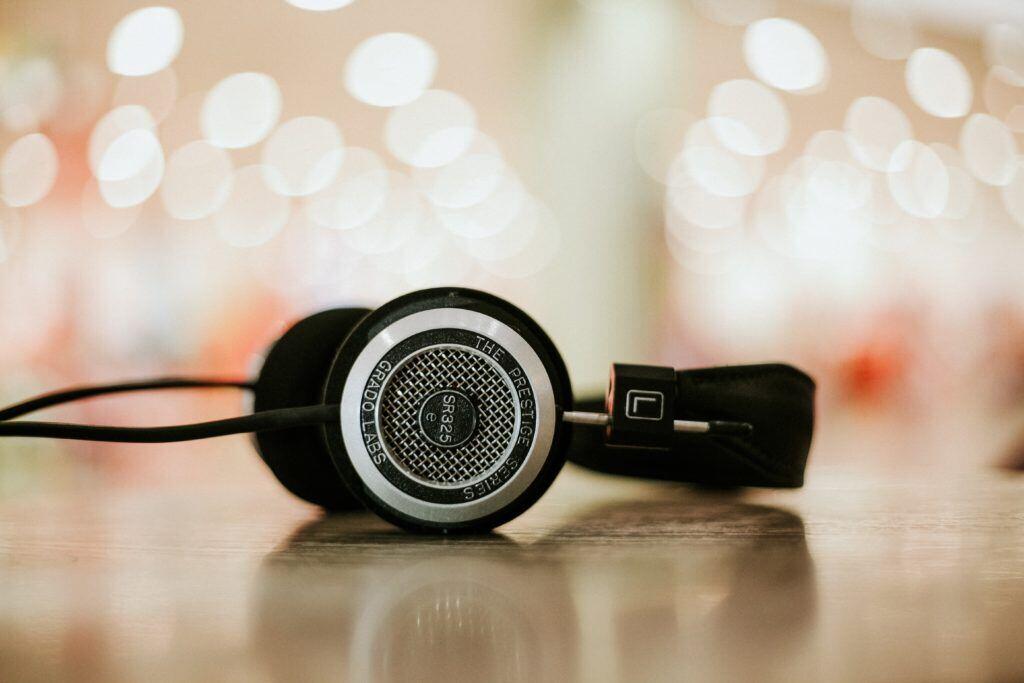
The Mozart Effect
Back in the early 1990s, researchers found that listening to Mozart’s “Sonata for Two Pianos in D Major” (K448) boosted the IQ and spatial reasoning skills of college students compared to those that did other relaxation techniques or nothing at all. This became known as " the Mozart effect," and it sparked curiosity among scientists. (It was even tested on rats---it turned out Mozart was quite a bit more helpful to maze-going rodents than minimalist composer Philip Glass was!)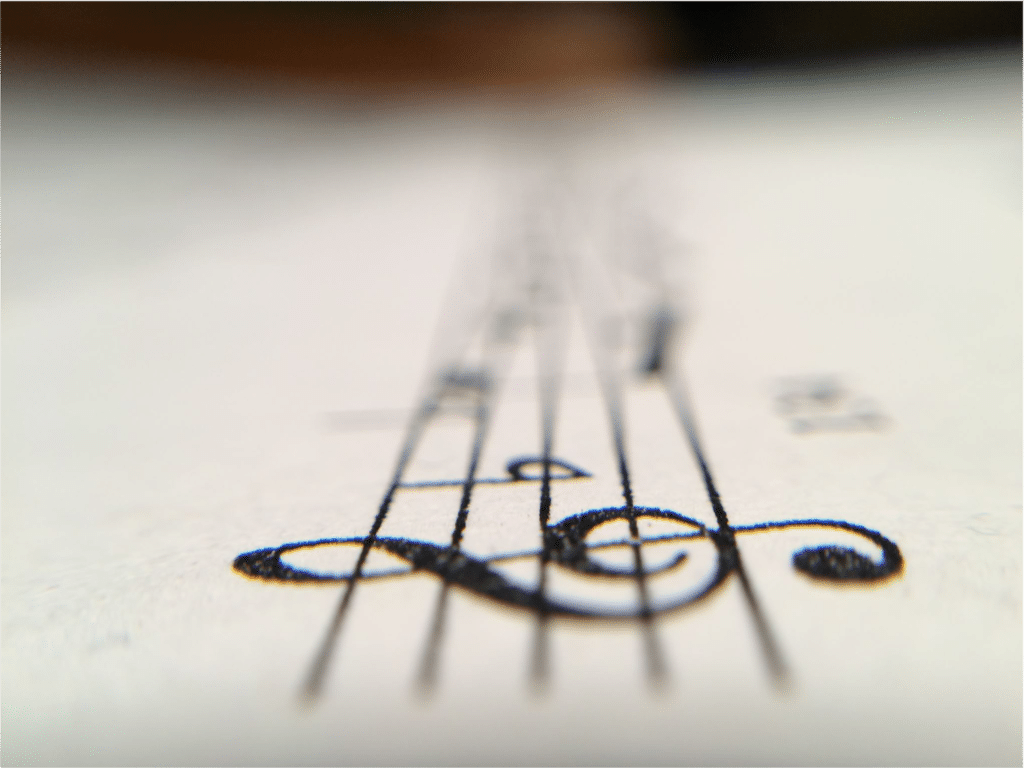
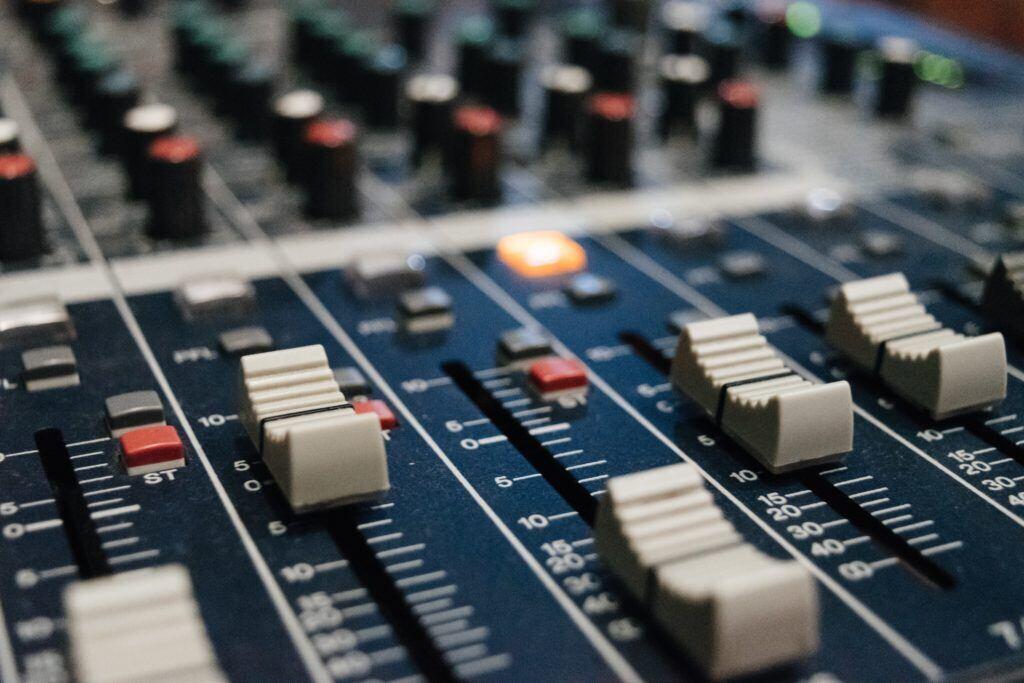
What Types of Algorithms are Applied in Machine Learning for Music Therapy?
[caption id="attachment_6678" align="aligncenter" width="668"]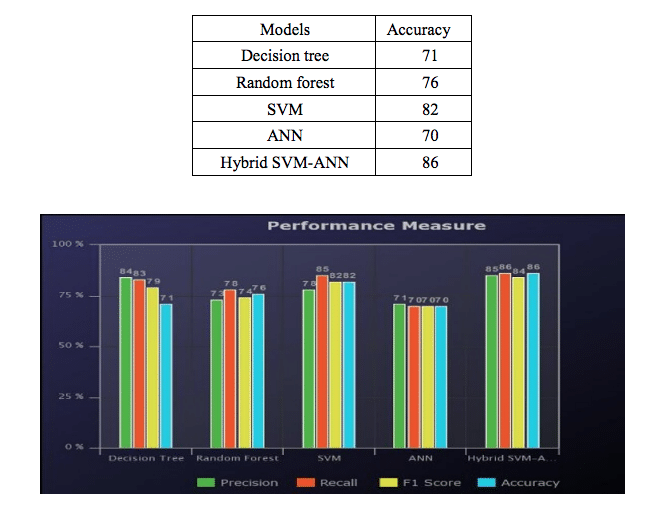
A Bright Future in Bright Melodies
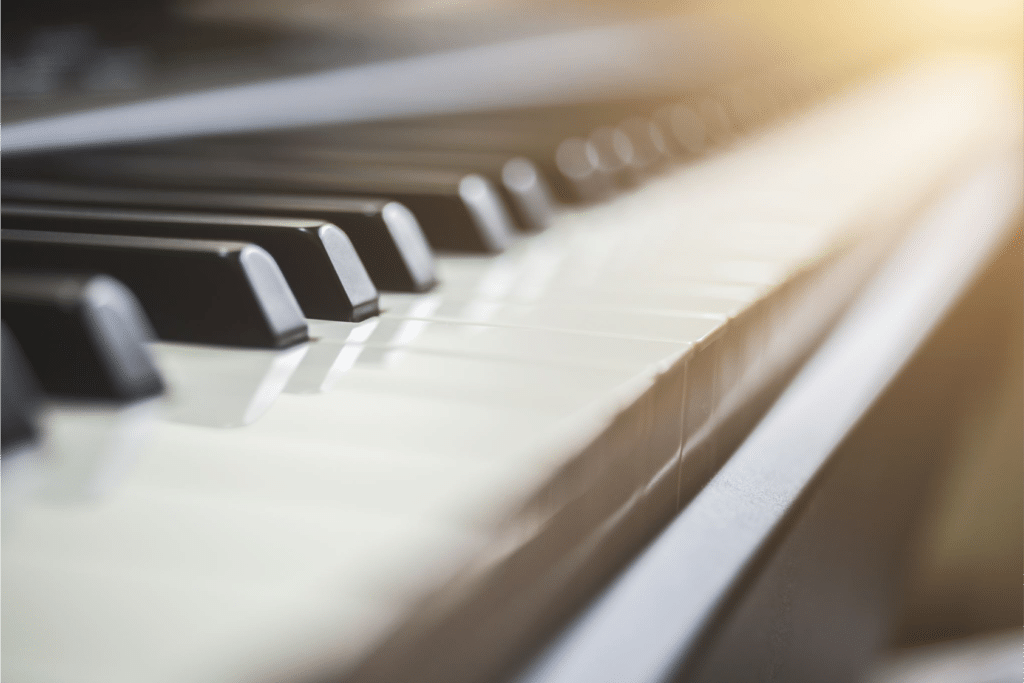
Stay connected. Join the Infused Innovations email list!
Share this
You May Also Like
These Related Posts
Technology Highlights of 2021
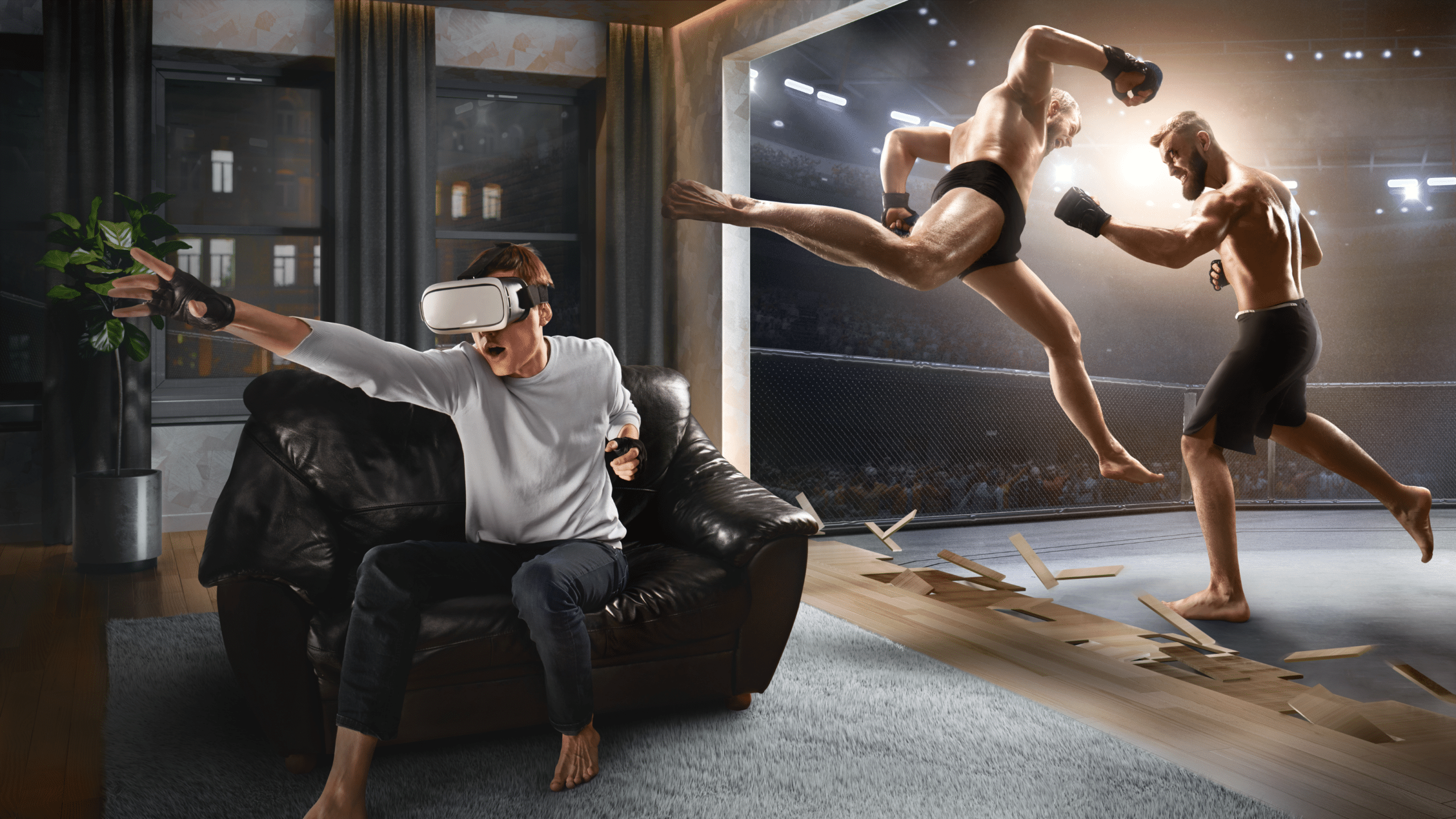
Technology Highlights of 2021
December 27, 2022
4
min read
3 Surprising Ways Technology Can Help Animals
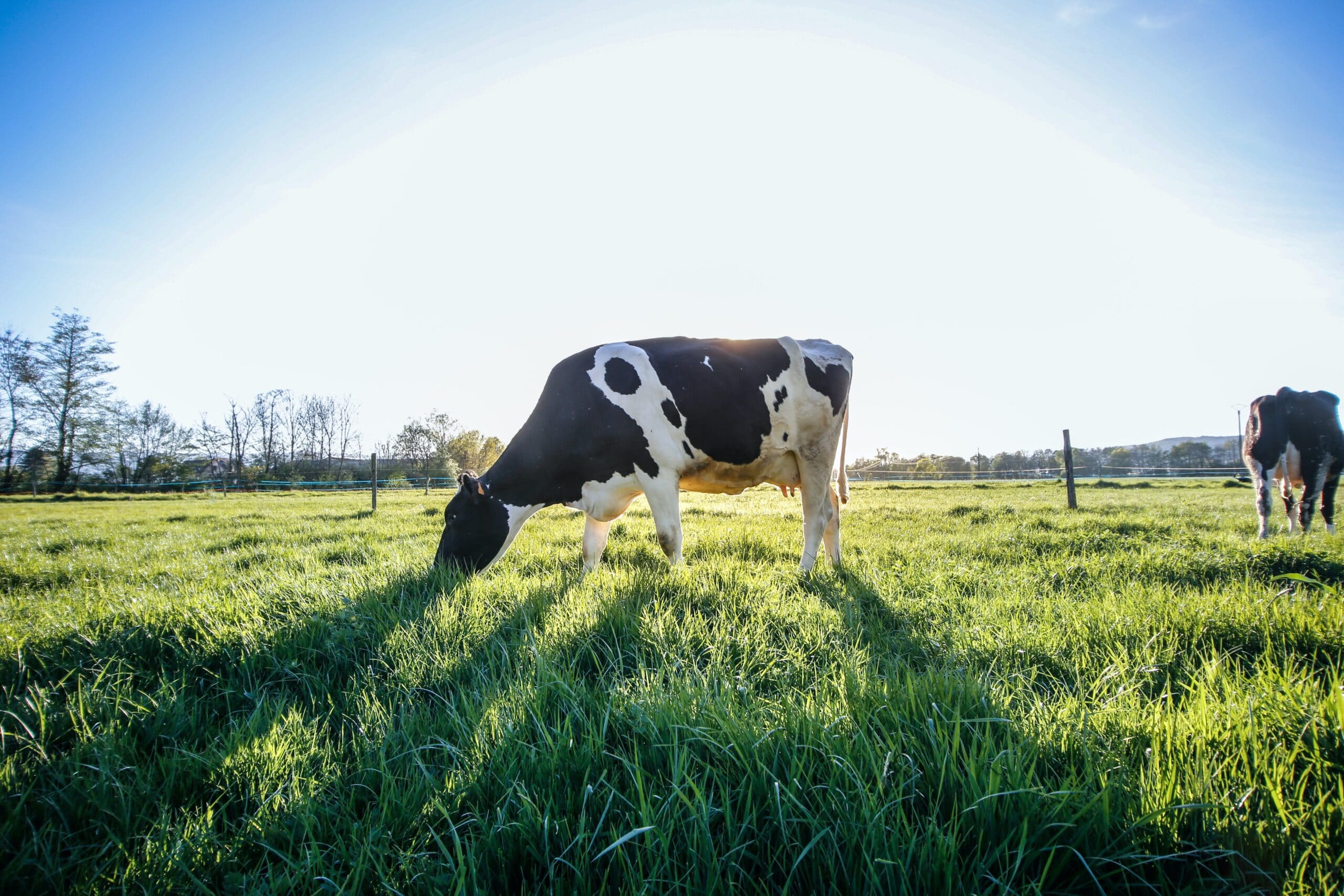
3 Surprising Ways Technology Can Help Animals
April 11, 2022
3
min read
Microsoft Defender for Endpoint and Intel TDT Combine for a New Ransomware Defense
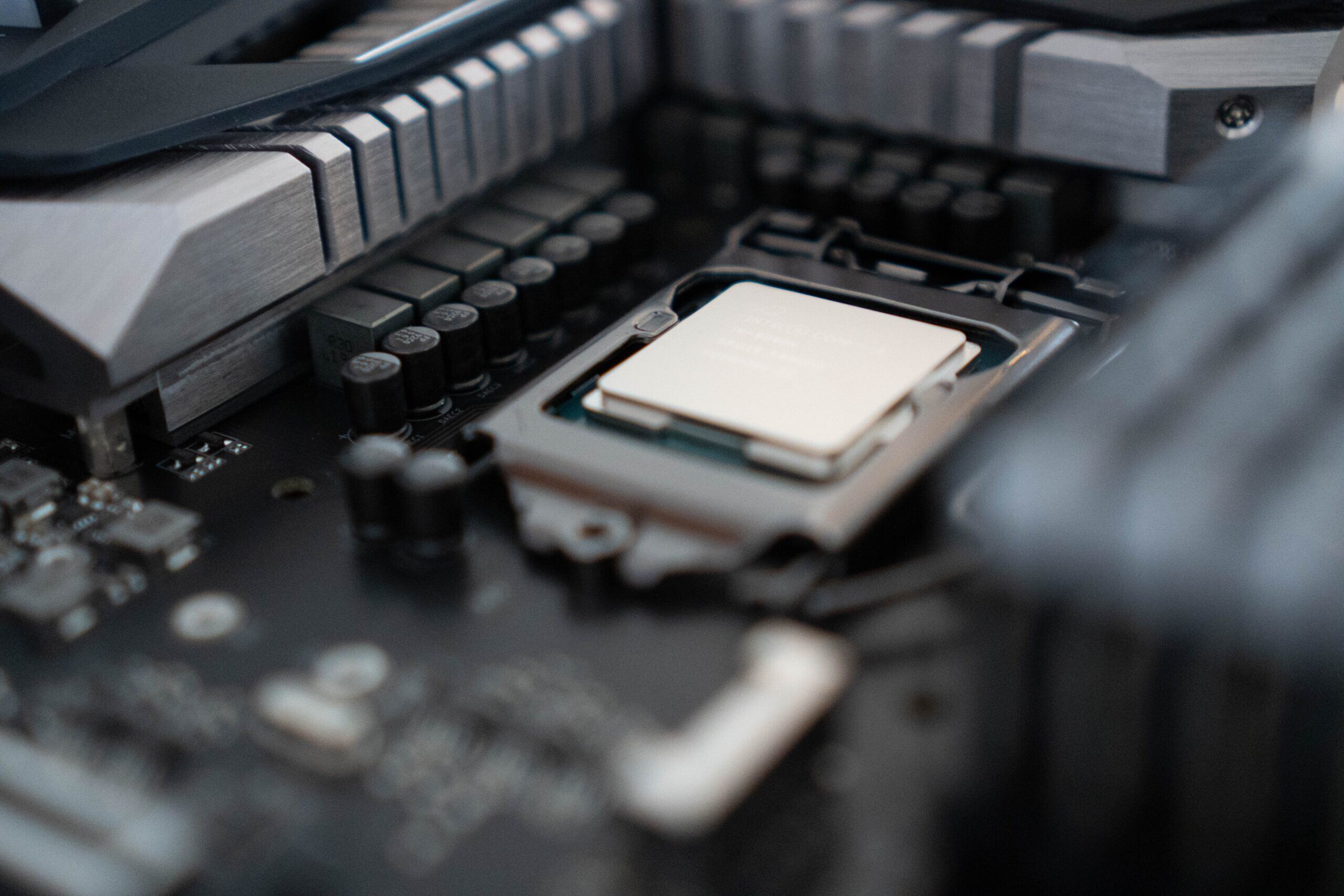
Microsoft Defender for Endpoint and Intel TDT Combine for a New Ransomware Defense
March 14, 2022
3
min read
No Comments Yet
Let us know what you think